-
Governance advisory
We guide boards and management teams in frameworks, team processes and leadership dynamics to deliver sustainable value.
-
Financial services advisory
Get market-driven expertise to achieve your goals in banking, insurance, capital markets, and investment management.
-
Business risk services
Our market-driven expertise helps firms keep growing and manage risk in an evolving regulatory landscape.
-
Risk
Meet risks with confidence and transform your business – we support you to manage risk and deliver on your goals.
-
Economic consulting
Bespoke guidance grounded in complex economic theory and practical sector insight to help you make the right decisions.
-
Government and public sector
Experience and expertise in delivering quality public sector advisory and audits.
-
Business consulting
Partnering with you to deliver sustainable business change that helps you realise your ambitions.
-
Transaction advisory services
Whether buying or selling, we help you get the deal done with our comprehensive range of transaction advisory services.
-
Financial accounting advisory services (FAAS)
Our FAAS team can support your finance function with the flexible resource they need to get results.
-
Corporate finance advisory
Building a business is never easy. We help you maximise the value of your business and find the right option.
-
Valuations
Help to understand or support the valuation of a business or asset.
-
Insolvency and global asset recovery
We provide asset tracing and seamless cross-border global recovery for clients.
-
Forensic and investigation services
Market-driven expertise in investigations, dispute resolution and digital forensics.
-
Restructuring
Our restructuring team help lenders, investors and management navigate contingency plans, restructuring and insolvency.
-
Transformation consulting
Is business transformation a priority for your organisation? Our expert insight and guidance can help you achieve it.
-
Pensions assurance
A tailored service that responds to evolving risks and regulations.
-
Accounting services
Optimise your growth with expert accounting services. Contact us today.
-
Royalty and intellectual property (IP) audits
Enhance IP asset protection with our royalty and IP audit services. Expertise in licensing, revenue detection, and compliance improvements.

-
Business consulting
Partnering with you to deliver sustainable business change that helps you realise your ambitions.
-
Corporate Simplification
Release value, reduce compliance complexity, and improve tax efficiency by streamlining your group structure.
-
Economic consulting
Bespoke guidance grounded in complex economic theory and practical sector insight to help you make the right decisions.
-
Financial accounting advisory services (FAAS)
Our FAAS team can support your finance function with the flexible resource they need to get results.
-
Governance advisory
We guide boards and management teams in frameworks, team processes and leadership dynamics to deliver sustainable value.
-
International
Unlock global opportunities with our local expertise and worldwide reach.
-
People advisory
Driving business performance through people strategy and culture.
-
Strategy Group
Successful business strategy is rooted in a clear understanding of the market, customer segmentation and how purchase decisions vary.
-
Respond: Data breach, incident response and computer forensics
Are you prepared for a cyber failure? We can help you avoid data breaches and offer support if the worst happens.
-
Comply: Cyber security regulation and compliance
Cyber security regulation and compliance is constantly evolving. Our team can support you through the digital landscape.
-
Protect: Cyber security strategy, testing and risk assessment
Cyber security threats are constantly evolving. We’ll work with you to develop and test robust people, process and technology defences to protect your data and information assets.
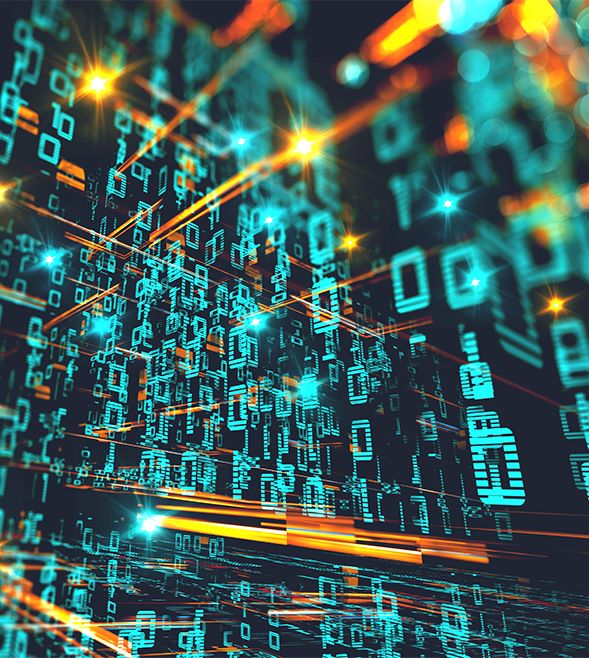
-
Corporate finance advisory
Building a business is never easy. We help you maximise the value of your business and find the right option.
-
Debt advisory
Working with borrowers and private equity financial sponsors on raising and refinancing debt. We can help you find the right lender and type of debt products.
-
Financial accounting advisory services (FAAS)
Our FAAS team can support your finance function with the flexible resource they need to get results.
-
Financial modelling services
Financial modelling that helps you wrestle with your most pressing business decisions.
-
Operational deal services
Enabling transaction goals through due diligence, integration, separation, and other complex change.
-
Our credentials
Search our transactions to see our experience in your sector and explore the deals advisory services we've delivered.
-
Transaction advisory services
Whether buying or selling, we help you get the deal done with our comprehensive range of transaction advisory services.
-
Valuations
Help to understand or support the valuation of a business or asset.
-
The ESG agenda
Shape your ESG agenda by identifying the right metrics, sustainable development and potential business value impact.
-
ESG driven business transition
Whatever your ESG strategy, we can support your organisation as it evolves while maximising efficiency and profitability.
-
ESG programme and change management
Do you have the right capabilities to drive the delivery of your ESG strategy to realise your targets?
-
ESG risk management
You must protect, comply, understand and influence to successfully manage the risk involved with ESG issues. We can help.
-
ESG strategy, risk and opportunity identification
We can help you clearly define your ESG Strategy, with the risks and opportunities identified and managed.
-
Create value through effective ESG communication
Building trust and engagement with your stakeholders on your ESG strategy.
-
ESG metrics, targets and disclosures
The pressure to report your ESG progress is growing. Do your targets measure up?
-
ESG governance, leadership and culture framework
Make the most of ESG opportunities by effectively embedding your strategy across your organisation.
-
ESG and non-financial assurance
Support your board to be confident in supplying robust information that withstands scrutiny.
-
Transition planning to net zero
Supporting your organisation in the transition to net zero.
-
Actuarial and insurance consulting
We consult extensively to the life insurance, general insurance, health insurance and pensions sectors.
-
Business risk services
Our market-driven expertise helps firms keep growing and manage risk in an evolving regulatory landscape.
-
Financial crime
Helping you fight financial crime in a constantly changing environment
-
Financial services business consulting
Leverage our diverse capabilities to manage challenges and take opportunities: from assurance to transformation
-
Financial services tax
Helping financial services firms navigate the global financial services and funds tax landscape.
-
Regulatory and compliance
Providing an exceptional level of regulatory and compliance to firms across the financial services industry.
-
Corporate intelligence
Corporate intelligence often involves cross-border complexities. Our experienced team can offer support.
-
Litigation support
Industry-wide litigation support and investigation services for lawyers and law firms.
-
Disputes advisory
Advising on quantum, accounting and financial issues in commercial disputes.
-
Forensic investigations and special situations
Do you need clarity in an uncertain situation? If you're accused of wrongdoing we can help you get the facts right.
-
Forensic data analytics
Our forensic data analytics team are helping businesses sift the truth from their data. See how we can help your firm.
-
Monitoring trustee and competition services
Monitoring trustee services to competition, financial and regulatory bodies.
-
Financial crime
Supporting your fight against financial crime in an ever-changing environment
-
Whistleblowing and investigation support
Whistleblowing frameworks provide many benefits – find out how to build trust and manage risks in a confidential, cost-effective, robust way.
-
Public sector advisory
To deliver excellent public services, local and central government need specialist support.
-
Public sector consulting
Helping public sector organisations maintain oversight of services and understand what's happening on the ground.
-
Public sector audit and assurance
As a leading UK auditor, we have unparalleled insights into the risks, challenges and opportunities that you face.
-
Competition damages and class actions
Helping you recover damages owed from anti-competitive practices.
-
Contentious estates and family disputes
We manage complex and sensitive disputes through to resolution.
-
Digital Asset Recovery
Get guidance and technical expertise on digital finance and cryptoasset recovery from our dedicated crypto hub.
-
Grant Thornton Offshore
Grant Thornton Offshore is our one-stop global solution for insolvency, asset recovery, restructuring and forensics services.
-
Insolvency Act Portal
Case information and published reports on insolvency cases handled by Grant Thornton UK LLP.
-
Litigation support
Industry-wide litigation support and investigation services for lawyers and law firms.
-
Personal insolvency
We can support you to maximise personal insolvency recovery and seek appropriate debt relief.
-
South Asia business group
We help Indian companies expand into the UK and invest globally. We also help UK companies invest and operate in India.
-
US business group
Optimise your trans-Atlantic operations with local knowledge and global reach.
-
Japan business group
Bridging the commercial and cultural divide and supporting your ambitions across Japan and the UK.
-
Africa business group
Connecting you to the right local teams in the UK, Africa, and the relevant offshore centres.
-
China-Britain business group
Supporting your operations across the China – UK economic corridor.
-
Asset based lending advisory
Helping lenders, their clients and other stakeholders navigate the complexities of ABL.
-
Contingency planning and administrations
In times of financial difficulty, it is vital that directors explore all the options that are available to them, including having a robust ‘Plan B’.
-
Corporate restructuring
Corporate restructuring can be a difficult time. Let our team make the process simple and as stress-free as possible.
-
Creditor and lender advisory
Whether you're a creditor or lender, complex restructurings depend on pragmatic commercial advice
-
Debt advisory
Our debt advisory team can find the right lender to help you in restructuring. Find out how our experts can support you.
-
Financial services restructuring and insolvency
Financial services restructuring and insolvency is a competitive marketplace. Our team can help you navigate this space.
-
Pensions advisory services
DB pension-schemes need a balanced approach that manages risk for trustees and sponsors in an uncertain economy.
-
Restructuring and insolvency tax
Tax will often be crucial in a plan to restructure a distressed business. Our team can guide you through the process.
-
Restructuring Plans
Market leading experience in advising companies and creditors in Restructuring Plan processes.
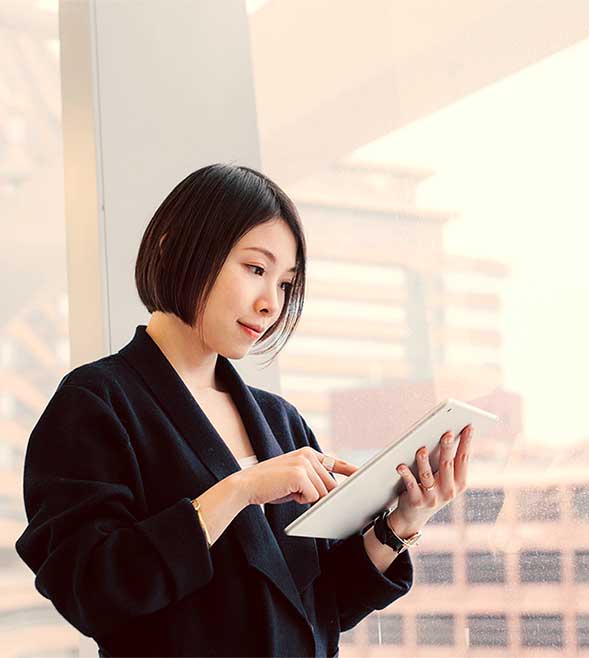
-
Artificial intelligence
Our approach to the design, development, and deployment of AI systems can assist with your compliance and regulation.
-
Controls advisory
Build a robust internal control environment in a changing world.
-
Data assurance and analytics
Enhancing your data processes, tools and internal capabilities to help you make decisions on managing risk and controls.
-
Enterprise risk management
Understand and embrace enterprise risk management – we help you develop and connect risk thinking to your objectives.
-
Internal audit services
Internal audit services that deliver the value and impact they should.
-
Managing risk and realising ESG opportunities
Assess and assure risk and opportunities across ESG with an expert, commercial and pragmatic approach.
-
Project, programme, and portfolio assurance
Successfully delivering projects and programmes include preparing for the wider impact on your business.
-
Service organisation controls report
Independent assurance provides confidence to your customers in relation to your services and control environment.
-
Supplier and contract assurance
Clarity around key supplier relationships: focusing on risk, cost, and operational performance.
-
Technology risk services
IT internal audits and technology risk assurance projects that help you manage your technology risks effectively.
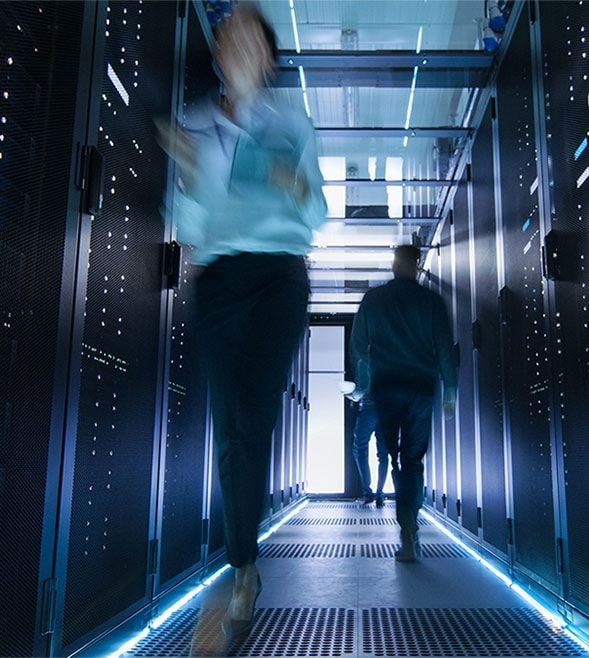
-
Capital allowances (tax depreciation)
Advisory and tools to help you realise opportunities in capital allowances.
-
Corporate tax
Helping companies manage corporate tax affairs: delivering actionable guidance to take opportunities and mitigate risk.
-
Employer solutions
We will help you deliver value through your employees, offering pragmatic employer solutions to increasing costs.
-
Financial services tax
Helping financial services firms navigate the global financial services and funds tax landscape.
-
Indirect tax
Businesses face complex ever changing VAT regimes, guidance and legislation. We can help you navigate these challenges.
-
International tax
Real-world international tax advice to help you navigate a changing global tax landscape.
-
Our approach to tax
We advise clients on tax law in the UK and, where relevant, other jurisdictions.
-
Private tax
Tax experts for entrepreneurs, families and private business. For now and the long term.
-
Real estate tax
Stay ahead of real estate tax changes with holistic, tax-efficient solutions.
-
Research and development tax incentives
We can help you prepare optimised and robust research and development tax claims.
-
Tax dispute resolution
We make it simple to stay compliant and avoid HMRC tax disputes
-
Tax risk management
We work with you to develop effective tax risk management strategies.
-
Skills and training
Get the right support to deliver corporate and vocational training that leads the way in an expanding market.
-
Private education
Insight and guidance for all businesses in the private education sector: from early years to higher education and edtech.
-
Facilities management and property services
Get insight and strategic support to take opportunities that protect resilience and drive UK and international growth.
-
Recruitment
Helping recruitment companies take opportunities to achieve their goals in a market where talent and skills are key.
-
Food and beverage (F&B)
We can help you find the right ingredients for growth in your food and beverage business.
-
Travel, tourism and leisure
Tap into our range of support for travel, tourism and leisure businesses in this period of challenge and change.
-
Retail, e-commerce and consumer products
With multiple challenges and opportunities in the fast-evolving retail sector, make sure you are ready for them.
-
Banking
Our expertise and insight can help you respond positively to long term and emerging issues in the banking sector.
-
Capital markets
2020 is a demanding year for capital markets. Working with you, we're architecting the future of the sector.
-
Insurance
Our experienced expert team brings you technical expertise and insight to guide you through insurance sector challenges.
-
Investment management
Embracing innovation and shaping business models for long-term success.
-
Pensions
Pension provision is an essential issue for employers, and the role of the trustee is becoming increasingly challenging.
-
Payments advisory and assurance
Payment service providers need to respond to rapidly evolving technical innovations and increased regulatory scrutiny.
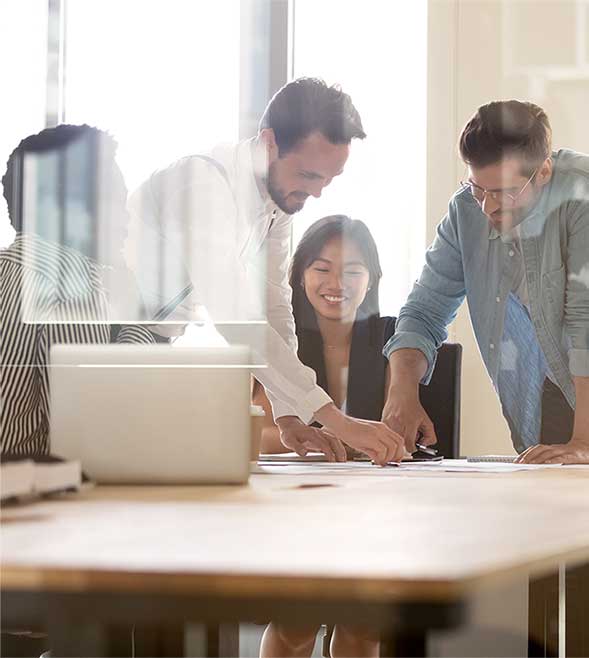
-
Central and devolved government
Helping central and devolved governments deliver change to improve our communities and grow our economies.
-
Infrastructure and transport
Delivering a successful transport or infrastructure project will require you to balance an often complex set of strategic issues.
-
Local government
Helping local government leverage technical and strategic expertise deliver their agendas and improve public services.
-
Regeneration development and housing
We provide commercial and strategic advice to assist your decision making in pursuing your objectives.
-
Health and social care
Sharing insight and knowledge to deliver transformation and improvement to health and social care services.
-
Charities
Supporting you to achieve positive change in the UK charity sector.
-
Education and skills
The education sector has rarely faced more risk or more opportunity to transform. You need to plan for the future.
-
Social housing
We are committed to helping change social housing for the better, and can help you make the most of every opportunity.

-
Technology
We work with dynamic technology companies of all sizes to help them succeed and grow internationally.
-
Telecommunications
Take all opportunities to realise your goals in telecommunications: from business refresh to international expansion.
-
Media
Media companies must stay agile to thrive in today’s highly competitive market – we’re here to support your ambitions.
-
Career development
The support you need will vary throughout your career, here are just some of the ways we'll support you to you excel with us.
-
How we work
Our approach to how we work, ensures that when we are making choices about how, where or when we work, we have the support and tools we need.
-
Reward and benefits
We are committed to building a culture where our people have access to the necessary benefits to help promote a healthy lifestyle and thrive.
-
Inclusion and diversity
Included and valued for your difference is how everyone should feel at work. Not just because it’s right, but because we’re all at our best when we’re able to be ourselves.
-
ESG: environment and community impact
Our ESG framework enables responsible, sustainable, and ethical operations. We prioritise the environment, our broader societal impact, and our firm's governance to protect the planet, foster inclusivity and wellbeing, support our communities, and bolster our firm's resilience.
-
What we do
It’s an exciting time to be joining Grant Thornton, especially as a trainee at the start of your career. Learn about our teams and the work we do.
-
Life as a trainee
Everything you need to know about life as a trainee, from the experiences you'll get to the skills you need.
-
Employability hub
Our employability hub is designed to help you feel prepared for the application process, and guide you through the decisions you will need to make throughout.
-
Our programmes
Whether you are looking to join us straight from school or with a degree, or even looking for some work experience, we have a programme that is right for you.
-
Parents, carers and teachers hub
Access information and support to help inform career discussions with your young people.
-
FAQs
We hope you can find all the information you need on our website, but to help we've collated a few of the questions we hear quite frequently when speaking to candidates.
-
Advisory
Our advisory practice provides organisations with the advice and solutions they need to unlock sustainable growth and navigate complex risks and challenges.
-
Audit
Every day our audit teams help people in businesses and communities to do what is right and achieve their goals.
-
Tax
The tax landscape is evolving, and our clients need us more than ever to navigate the complexities with them.
-
Central Client Service
From finance and information systems, to marketing and people teams we have a diverse range of teams supporting our business.
-
Our recruitment process
We strive to ensure our interview process is barrier free and sets you up for success, as well as being wholly inclusive and robust. Learn about our process here.
-
International hiring
Our international talent is an important part of our firm, joining us you will be joining a growing community of international hires.
-
Armed forces
As an Armed Forces Friendly organisation we are proud to support members of the Armed Forces Community as they develop their career outside of the Armed Forces.
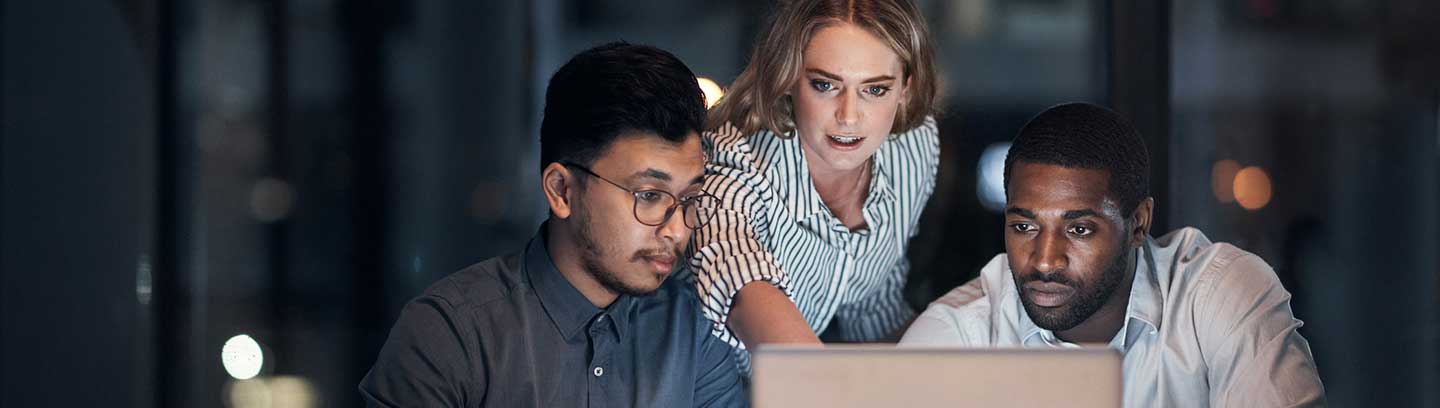
The financial services sector remains a high-profile target for cyber criminals, and the threat landscape is increasingly complex with the use of AI-powered cyber-attacks. As such, many firms are asking their cyber security teams to match these activities and make good use of AI for defensive purposes. But it’s not always clear where to start, partly because many firms don’t have the necessary skills to identify potential use cases or, where these use cases are clear, to put them into practice. Keeping pace with the evolving threat landscape is essential, or firms will face greater cyber threat exposure, risking customer data and increasing the potential for service outages.
Meeting regulatory requirements
Successful cyber-attacks are a significant problem for all organisations, with expensive recovery work and lasting reputational damage. For the financial sector, there’s also operational resilience to consider and firms need to be able to restore outages within pre-defined tolerance limits. While the onus is on restoring services promptly, that doesn’t absolve firms of their need to actively prevent cyber breaches. This is also important in the context of Consumer Duty, where a successful attack due to poor cyber risk management could result in customer harm, and lead to the implementation of remedial plans to compensate customers for their loss and distress.
Financial services firms need to use all available tools, including AI, to strengthen their cyber security posture and demonstrate an appropriate control environment. This includes both effective cyber risk management and a good control framework for the use of AI itself (including robust oversight and challenge over AI use by third-party organisations).
Threat intelligence and predictions
AI can proactively identify potential cyber risks by analysing both global intelligence and firm-specific data for a more cohesive view of the threat landscape. Natural language processing (NLP) can assess dark web sources, cybersecurity reports and industry databases for greater horizon scanning and signal detection. AI algorithms can also analyse patterns from past incidents to determine potential attack vectors.
Meanwhile, forward-looking models can assess the likelihood of specific threats (such as ransomware or phishing) based on historical data, behavioural indicators and real-time analysis. Firms can also apply learning models to detect emerging trends or vulnerabilities specific to the organisation’s own infrastructure.
Drawing on the above, AI can then assign risk-scores and prioritise potential threats, considering their sophistication, impact and likelihood. This can help firms allocate resources towards high-priority areas and free up skilled personnel to focus on more challenging activities.
AI-driven detection and monitoring
Perhaps the most mature use of AI in cyber security is real-time threat and anomaly detection across networks and systems. Machine learning models can monitor network traffic and reduce the potential for distributed denial-of-service (DDoS) attacks and data exfiltration. Firms can implement user and entity behaviour analytics (UEBA) to identify where an account or application is acting in an unusual way, indicating that it could compromised. This is further supported by AI to detect suspicious activity on end-user terminals, such as laptops or mobiles, and Internet of Things (IoT) devices.
Automated learning, behavioural modelling and anomaly detection in Privileged Access Management also allows firms to proactively identify inappropriate access to confidential information. These types of detections are vital, with Crowdstrike’s 2025 Global Threat Report highlighting that up to 79% of attacks it detected in 2024 were achieved without malware and were typically the result of compromised accounts.
While the above are powerful tools in their own right, firms can combine them with identity and access management systems (IAMs) to automatically adjust permissions and flag exceptions in real-time.
Automated incident response and recovery
In the event of a cyber breach, firms need to secure their systems and restore services promptly, in line with operational resilience obligations. While most financial services firms will have an incident response playbook, many are missing opportunities to automate those actions based on pre-set trigger events. Automated responses include blocking malicious IP addresses, locking compromised accounts or isolating infected devices. Taking these practices a step further, incident response teams can use decision-tree AI models to base actions on incident context and regulatory obligations.
Intelligent threat containment is a key element of a modern cyber-resilience approach, using AI to identify the affected systems, determine attack spread, and proactively isolate critical assets while avoiding operational disruptions. Good integration with security orchestration, automation and response (SOAR) tools can automatically apply pre-defined remediation protocols, speeding up cyber incident response times.
Turing our attention to cyber incident recovery, AI-based analytics can carry out root cause analysis to identifying the attack vectors used and the vulnerabilities exploited. NLP tools can further support this area by generating comprehensive incident reports for compliance, including insights on attack patterns and suggested preventive measures moving forward.
Boosting training and awareness
Good use of AI can support robust cyber security training, simulate attacks and reinforce a good security culture. Phishing remains one of the most common attack vectors, and firms can use AI to generate personalised phishing simulations, based on known tactics and real-world threats.
Combing this approach with behavioural analysis can identify individuals who may be at higher risk of security breaches, for example those working with sensitive data, and tailor training campaigns accordingly. Cyber criminals often weaponise AI-powered approaches to identify soft targets and ‘whales’ – high-profile targets for spear phishing. Firms can use the same technologies to get ahead of the risks, using AI to curate interactive security awareness programs that provide real-time feedback to reinforce good practices and increase engagement.
Continuous learning and threat adaptation
Good cyber security is an ongoing process, and firms need to continually retrain and fine-tune their AI models with new data to make sure they’re up to date. This includes adversarial machine learning to test AI models against simulated cyber-attacks and emerging threats. Firms can also draw on post-incident reviews, insights from industry-wide forums and regulatory bodies, and shared data to improve AI effectiveness.
In the rush to move forward, it’s also important to be aware of threats from within the AI model itself. There have already been instances of poisoned AI models being deployed in commercial settings. They have in-built backdoors and vulnerabilities for attackers to take advantage of, including breaking out of the data silos used for training purposes. The AI can then be used as a new attack-surface for cyber criminals, and many businesses may benefit from expert support to build and deploy them safely.
Regulatory compliance and reporting automation
AI can help firms maintain regulatory compliance, including cyber and data-focused regulations such as the Payment Card Industry Data Security Standard (PCI-DSS) and the General Data Protection Regulation (GDPR). Machine learning algorithms can enable risk management dashboards to flag exceptions in real time, streamline reporting processes and reduce the potential for regulatory breaches.
As the regulatory landscape evolves, NLP can also scan for new guidance and automatically adjust compliance settings. However, as with all aspects of AI and machine learning, it’s essential to make sure there is appropriate governance and oversight in place to sense-check those activities.
Getting started with AI in cyber security
As its use becomes more widespread, AI is becoming an increasingly important element of the cyber threat landscape. Firms that continue to rely on manual cyber security processes may struggle to keep pace with AI-powered attacks, increasing the potential for a security breach. Taking early action to embed AI in cyber security can make a significant impact to all financial services firms and support regulatory compliance. However, it relies on good leadership and clear alignment with wider organisational goals.
To get started, firms can create a cyber risk committee of key stakeholders – including senior executives, IT heads, data scientists and compliance leads – to define AI’s role in cybersecurity. This includes creating AI policies over usage, key constraints, data privacy and alignment with regulatory standards, such as Consumer Duty, operational resilience and GDPR.
The preferred approach will need to align with the firm’s broader objectives, risk appetite and risk management frameworks. As with any other risk management approach, it’s vital to embed effective governance processes, establish metrics for success and track performance. This will support continual improvement plans and help maintain a robust cyber security environment in the long term.
For insight and guidance on the use of AI in cyber security for financial services, contact Manu Sharma or Miles Davis.
