-
Governance advisory
We guide boards and management teams in frameworks, team processes and leadership dynamics to deliver sustainable value.
-
Financial services advisory
Get market-driven expertise to achieve your goals in banking, insurance, capital markets, and investment management.
-
Business risk services
Our market-driven expertise helps firms keep growing and manage risk in an evolving regulatory landscape.
-
Risk
Meet risks with confidence and transform your business – we support you to manage risk and deliver on your goals.
-
Economic consulting
Bespoke guidance grounded in complex economic theory and practical sector insight to help you make the right decisions.
-
Government and public sector
Experience and expertise in delivering quality public sector advisory and audits.
-
Business consulting
Partnering with you to deliver sustainable business change that helps you realise your ambitions.
-
Transaction advisory services
Whether buying or selling, we help you get the deal done with our comprehensive range of transaction advisory services.
-
Financial accounting advisory services (FAAS)
Our FAAS team can support your finance function with the flexible resource they need to get results.
-
Corporate finance advisory
Building a business is never easy. We help you maximise the value of your business and find the right option.
-
Valuations
Help to understand or support the valuation of a business or asset.
-
Insolvency and global asset recovery
We provide asset tracing and seamless cross-border global recovery for clients.
-
Forensic and investigation services
Market-driven expertise in investigations, dispute resolution and digital forensics.
-
Restructuring
Our restructuring team help lenders, investors and management navigate contingency plans, restructuring and insolvency.
-
Transformation consulting
Is business transformation a priority for your organisation? Our expert insight and guidance can help you achieve it.
-
Pensions assurance
A tailored service that responds to evolving risks and regulations.
-
Accounting services
Optimise your growth with expert accounting services. Contact us today.
-
Royalty and intellectual property (IP) audits
Enhance IP asset protection with our royalty and IP audit services. Expertise in licensing, revenue detection, and compliance improvements.
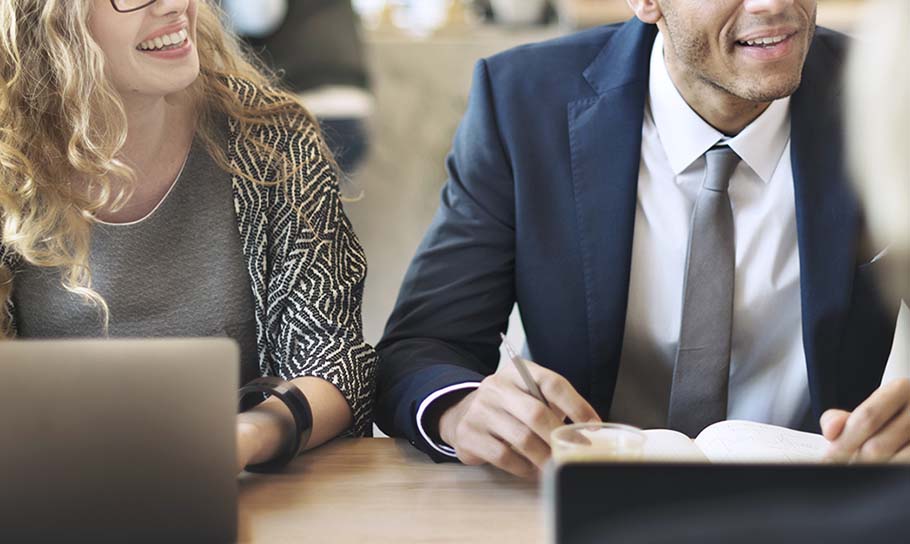
-
Business consulting
Partnering with you to deliver sustainable business change that helps you realise your ambitions.
-
Corporate Simplification
Release value, reduce compliance complexity, and improve tax efficiency by streamlining your group structure.
-
Economic consulting
Bespoke guidance grounded in complex economic theory and practical sector insight to help you make the right decisions.
-
Financial accounting advisory services (FAAS)
Our FAAS team can support your finance function with the flexible resource they need to get results.
-
Governance advisory
We guide boards and management teams in frameworks, team processes and leadership dynamics to deliver sustainable value.
-
International
Unlock global opportunities with our local expertise and worldwide reach.
-
People advisory
Driving business performance through people strategy and culture.
-
Strategy Group
Successful business strategy is rooted in a clear understanding of the market, customer segmentation and how purchase decisions vary.
-
Respond: Data breach, incident response and computer forensics
Are you prepared for a cyber failure? We can help you avoid data breaches and offer support if the worst happens.
-
Comply: Cyber security regulation and compliance
Cyber security regulation and compliance is constantly evolving. Our team can support you through the digital landscape.
-
Protect: Cyber security strategy, testing and risk assessment
Cyber security threats are constantly evolving. We’ll work with you to develop and test robust people, process and technology defences to protect your data and information assets.
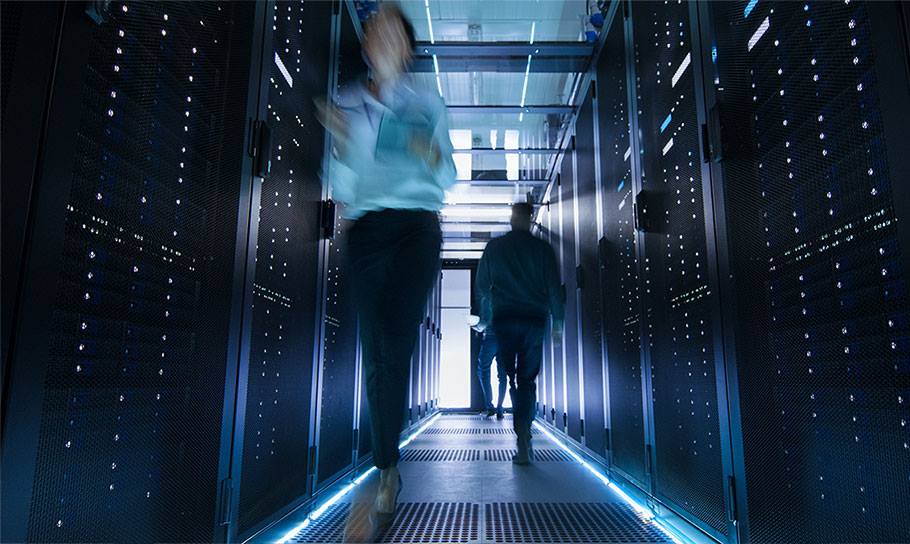
-
Corporate finance advisory
Building a business is never easy. We help you maximise the value of your business and find the right option.
-
Debt advisory
Working with borrowers and private equity financial sponsors on raising and refinancing debt. We can help you find the right lender and type of debt products.
-
Financial accounting advisory services (FAAS)
Our FAAS team can support your finance function with the flexible resource they need to get results.
-
Financial modelling services
Financial modelling that helps you wrestle with your most pressing business decisions.
-
Operational deal services
Enabling transaction goals through due diligence, integration, separation, and other complex change.
-
Our credentials
Search our transactions to see our experience in your sector and explore the deals advisory services we've delivered.
-
Transaction advisory services
Whether buying or selling, we help you get the deal done with our comprehensive range of transaction advisory services.
-
Valuations
Help to understand or support the valuation of a business or asset.
-
The ESG agenda
Shape your ESG agenda by identifying the right metrics, sustainable development and potential business value impact.
-
ESG driven business transition
Whatever your ESG strategy, we can support your organisation as it evolves while maximising efficiency and profitability.
-
ESG programme and change management
Do you have the right capabilities to drive the delivery of your ESG strategy to realise your targets?
-
ESG risk management
You must protect, comply, understand and influence to successfully manage the risk involved with ESG issues. We can help.
-
ESG strategy, risk and opportunity identification
We can help you clearly define your ESG Strategy, with the risks and opportunities identified and managed.
-
Create value through effective ESG communication
Building trust and engagement with your stakeholders on your ESG strategy.
-
ESG metrics, targets and disclosures
The pressure to report your ESG progress is growing. Do your targets measure up?
-
ESG governance, leadership and culture framework
Make the most of ESG opportunities by effectively embedding your strategy across your organisation.
-
ESG and non-financial assurance
Support your board to be confident in supplying robust information that withstands scrutiny.
-
Transition planning to net zero
Supporting your organisation in the transition to net zero.
-
Actuarial and insurance consulting
We consult extensively to the life insurance, general insurance, health insurance and pensions sectors.
-
Business risk services
Our market-driven expertise helps firms keep growing and manage risk in an evolving regulatory landscape.
-
Financial crime
Helping you fight financial crime in a constantly changing environment
-
Financial services business consulting
Leverage our diverse capabilities to manage challenges and take opportunities: from assurance to transformation
-
Financial services tax
Helping financial services firms navigate the global financial services and funds tax landscape.
-
Regulatory and compliance
Providing an exceptional level of regulatory and compliance to firms across the financial services industry.
-
Corporate intelligence
Corporate intelligence often involves cross-border complexities. Our experienced team can offer support.
-
Litigation support
Industry-wide litigation support and investigation services for lawyers and law firms.
-
Disputes advisory
Advising on quantum, accounting and financial issues in commercial disputes.
-
Forensic investigations and special situations
Do you need clarity in an uncertain situation? If you're accused of wrongdoing we can help you get the facts right.
-
Forensic data analytics
Our forensic data analytics team are helping businesses sift the truth from their data. See how we can help your firm.
-
Monitoring trustee and competition services
Monitoring trustee services to competition, financial and regulatory bodies.
-
Financial crime
Supporting your fight against financial crime in an ever-changing environment
-
Public sector advisory
To deliver excellent public services, local and central government need specialist support.
-
Public sector consulting
Helping public sector organisations maintain oversight of services and understand what's happening on the ground.
-
Public sector audit and assurance
As a leading UK auditor, we have unparalleled insights into the risks, challenges and opportunities that you face.
-
Contentious estates and family disputes
We manage complex and sensitive disputes through to resolution.
-
Digital Asset Recovery
Get guidance and technical expertise on digital finance and cryptoasset recovery from our dedicated crypto hub.
-
Grant Thornton Offshore
Grant Thornton Offshore is our one-stop global solution for insolvency, asset recovery, restructuring and forensics services.
-
Insolvency Act Portal
Case information and published reports on insolvency cases handled by Grant Thornton UK LLP.
-
Litigation support
Industry-wide litigation support and investigation services for lawyers and law firms.
-
Personal insolvency
We can support you to maximise personal insolvency recovery and seek appropriate debt relief.
-
South Asia business group
We help Indian companies expand into the UK and invest globally. We also help UK companies invest and operate in India.
-
US business group
Optimise your trans-Atlantic operations with local knowledge and global reach.
-
Japan business group
Bridging the commercial and cultural divide and supporting your ambitions across Japan and the UK.
-
Africa business group
Connecting you to the right local teams in the UK, Africa, and the relevant offshore centres.
-
China-Britain business group
Supporting your operations across the China – UK economic corridor.
-
Asset based lending advisory
Helping lenders, their clients and other stakeholders navigate the complexities of ABL.
-
Contingency planning and administrations
In times of financial difficulty, it is vital that directors explore all the options that are available to them, including having a robust ‘Plan B’.
-
Corporate restructuring
Corporate restructuring can be a difficult time. Let our team make the process simple and as stress-free as possible.
-
Creditor and lender advisory
Whether you're a creditor or lender, complex restructurings depend on pragmatic commercial advice
-
Debt advisory
Our debt advisory team can find the right lender to help you in restructuring. Find out how our experts can support you.
-
Financial services restructuring and insolvency
Financial services restructuring and insolvency is a competitive marketplace. Our team can help you navigate this space.
-
Pensions advisory services
DB pension-schemes need a balanced approach that manages risk for trustees and sponsors in an uncertain economy.
-
Restructuring and insolvency tax
Tax will often be crucial in a plan to restructure a distressed business. Our team can guide you through the process.
-
Restructuring Plans
Market leading experience in advising companies and creditors in Restructuring Plan processes.
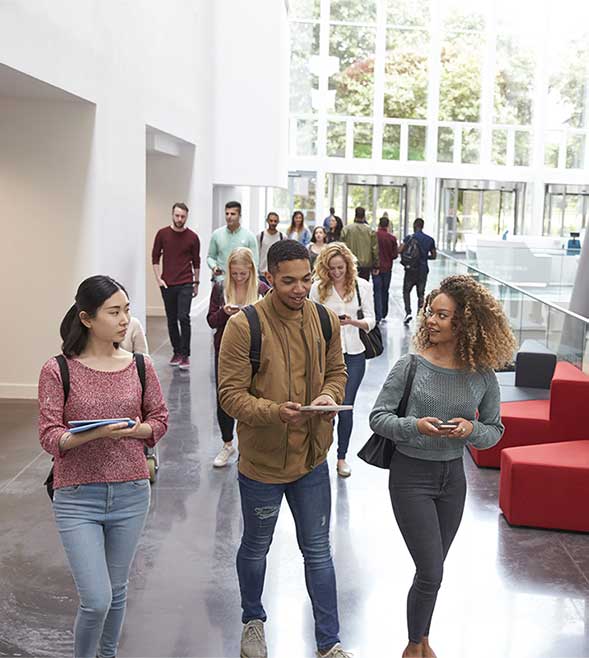
-
Controls advisory
Build a robust internal control environment in a changing world.
-
Data assurance and analytics
Enhancing your data processes, tools and internal capabilities to help you make decisions on managing risk and controls.
-
Enterprise risk management
Understand and embrace enterprise risk management – we help you develop and connect risk thinking to your objectives.
-
Internal audit services
Internal audit services that deliver the value and impact they should.
-
Managing risk and realising ESG opportunities
Assess and assure risk and opportunities across ESG with an expert, commercial and pragmatic approach.
-
Project, programme, and portfolio assurance
Successfully delivering projects and programmes include preparing for the wider impact on your business.
-
Service organisation controls report
Independent assurance provides confidence to your customers in relation to your services and control environment.
-
Supplier and contract assurance
Clarity around key supplier relationships: focusing on risk, cost, and operational performance.
-
Technology risk services
IT internal audits and technology risk assurance projects that help you manage your technology risks effectively.
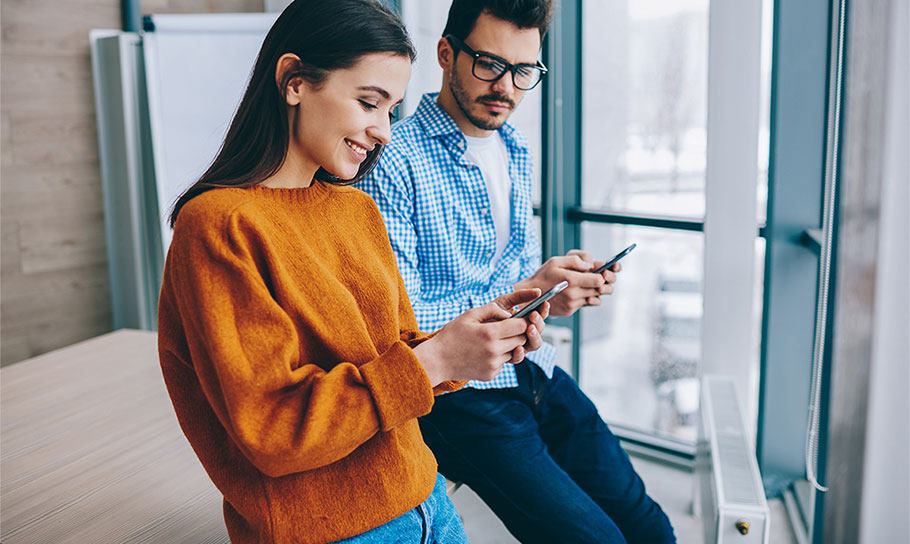
-
Capital allowances (tax depreciation)
Advisory and tools to help you realise opportunities in capital allowances.
-
Corporate tax
Helping companies manage corporate tax affairs: delivering actionable guidance to take opportunities and mitigate risk.
-
Employer solutions
We will help you deliver value through your employees, offering pragmatic employer solutions to increasing costs.
-
Indirect tax
Businesses face complex ever changing VAT regimes, guidance and legislation. We can help you navigate these challenges.
-
International tax
Real-world international tax advice to help you navigate a changing global tax landscape.
-
Our approach to tax
We advise clients on tax law in the UK and, where relevant, other jurisdictions.
-
Private tax
Tax experts for entrepreneurs, families and private business. For now and the long term.
-
Real estate tax
Stay ahead of real estate tax changes with holistic, tax-efficient solutions.
-
Research and development tax incentives
We can help you prepare optimised and robust research and development tax claims.
-
Tax dispute resolution
We make it simple to stay compliant and avoid HMRC tax disputes
-
Tax risk management
We work with you to develop effective tax risk management strategies.
-
Skills and training
Get the right support to deliver corporate and vocational training that leads the way in an expanding market.
-
Private education
Insight and guidance for all businesses in the private education sector: from early years to higher education and edtech.
-
Facilities management and property services
Get insight and strategic support to take opportunities that protect resilience and drive UK and international growth.
-
Recruitment
Helping recruitment companies take opportunities to achieve their goals in a market where talent and skills are key.
-
Food and beverage (F&B)
We can help you find the right ingredients for growth in your food and beverage business.
-
Travel, tourism and leisure
Tap into our range of support for travel, tourism and leisure businesses in this period of challenge and change.
-
Retail, e-commerce and consumer products
With multiple challenges and opportunities in the fast-evolving retail sector, make sure you are ready for them.
-
Banking
Our expertise and insight can help you respond positively to long term and emerging issues in the banking sector.
-
Capital markets
2020 is a demanding year for capital markets. Working with you, we're architecting the future of the sector.
-
Insurance
Our experienced expert team brings you technical expertise and insight to guide you through insurance sector challenges.
-
Investment management
Embracing innovation and shaping business models for long-term success.
-
Pensions
Pension provision is an essential issue for employers, and the role of the trustee is becoming increasingly challenging.
-
Payments advisory and assurance
Payment service providers need to respond to rapidly evolving technical innovations and increased regulatory scrutiny.
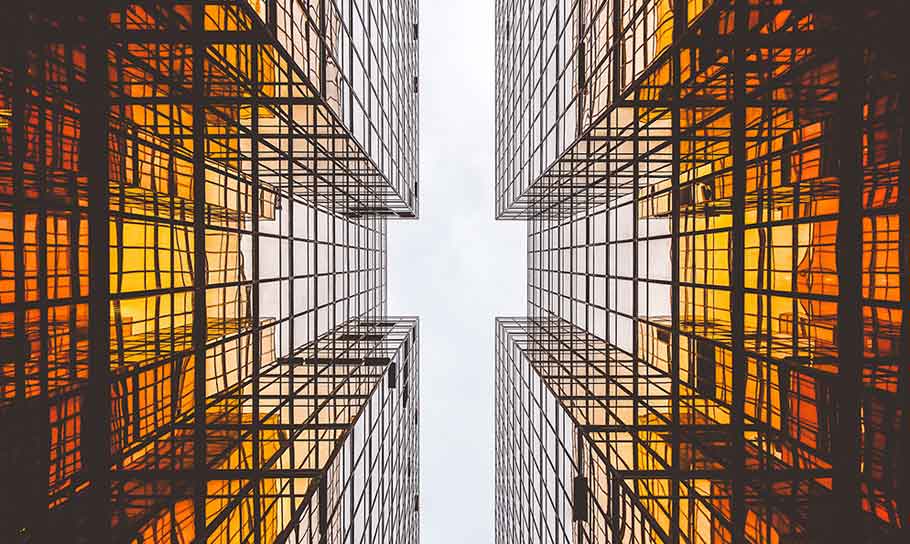
-
Central and devolved government
Helping central and devolved governments deliver change to improve our communities and grow our economies.
-
Infrastructure and transport
Delivering a successful transport or infrastructure project will require you to balance an often complex set of strategic issues.
-
Local government
Helping local government leverage technical and strategic expertise deliver their agendas and improve public services.
-
Regeneration development and housing
We provide commercial and strategic advice to assist your decision making in pursuing your objectives.
-
Health and social care
Sharing insight and knowledge to deliver transformation and improvement to health and social care services.
-
Charities
Supporting you to achieve positive change in the UK charity sector.
-
Education and skills
The education sector has rarely faced more risk or more opportunity to transform. You need to plan for the future.
-
Social housing
We are committed to helping change social housing for the better, and can help you make the most of every opportunity.
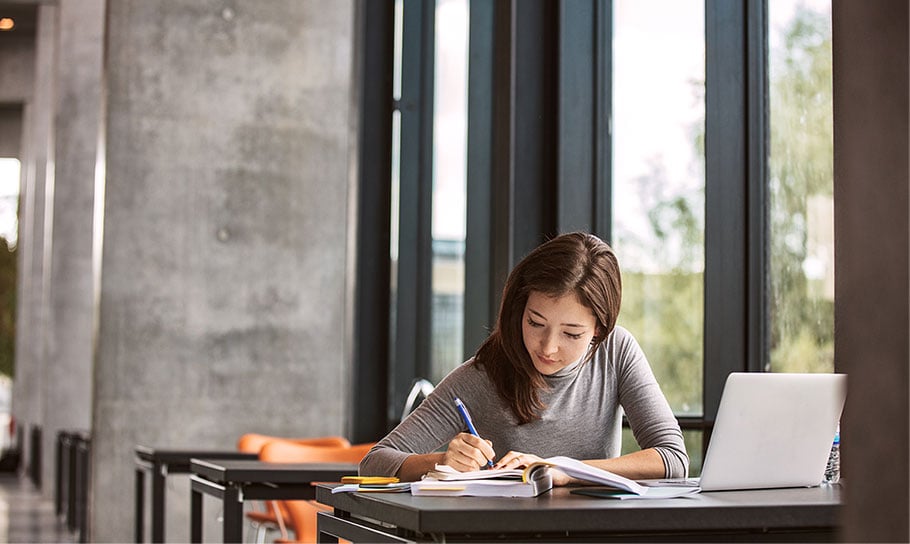
-
Technology
We work with dynamic technology companies of all sizes to help them succeed and grow internationally.
-
Telecommunications
Take all opportunities to realise your goals in telecommunications: from business refresh to international expansion.
-
Media
Media companies must stay agile to thrive in today’s highly competitive market – we’re here to support your ambitions.
What risks can data mitigate?
The current economic turndown is putting multiple pressures on business: inflation, rising interest rates, and labour shortages. You can measure the impact of these changing conditions through monitoring key risk indicators. These metrics use both internal and external data to provide insight into emerging risk conditions and show what your business needs to do to mitigate those risks.
Product or customer diversification
If one aspect of your business experiences a decline, analysing product or customer diversification will help to quantify any single points of failure, and provide opportunities for expanding into new markets or customers.
Be agile and prioritise essential products
Adapting your business strategy to evolving market conditions can help you sustain success. Analysis of product sales and margins will highlight which products are loss-making and not contributing to cash flow. This can be monitored on an ongoing basis to ensure changing input and delivery costs are reflected on a timely basis.
Leverage predictive analytics
During an economic downturn, predictive analytics can be a potent asset in foreseeing market trends and pinpointing avenues for expansion. Analysing purchasing data and demographic information, businesses can develop targeted marketing campaigns to customers, offering them promotions and discounts to keep them engaged with your brand.
Fraud risk mitigation
The risk of fraud tends to increase during a recession, but data analytics can play a crucial role in detecting it by examining large volumes of data to identify suspicious patterns or anomalies.
Anomaly detection
By establishing baseline patterns of normal behaviour, data analytics can identify anomalies that may indicate fraudulent activities. Unusual transactions, unexpected changes in customer behaviour, or abnormal patterns in financial data can be flagged for further investigation.
Predictive modelling
Utilising historical data and machine learning algorithms, predictive modelling can identify patterns that are indicative of fraudulent behaviour. These models can be used to score transactions or activities based on their likelihood of being fraudulent, so you can prioritise investigations.
Real-time monitoring
By implementing real-time data analytics systems, organisations can continuously monitor transactions and activities, promptly detecting and responding to potential fraud. Real-time alerts can be triggered based on predefined rules or anomalous behaviour patterns.
Text and sentiment analysis
Data analytics techniques can be applied to text data, such as emails, customer reviews, or social media posts, to identify potentially fraudulent activities or indicators. Sentiment analysis can uncover negative sentiment or unusual language patterns associated with fraudulent behaviour.
Behavioural analysis
Data analytics can analyse user behaviour, such as navigation patterns, login activities, or transaction histories, to detect deviations from normal behaviour. Unusual behaviour or access patterns can raise red flags and prompt further investigation.
Cost controls
Maintaining financial stability for your business can be achieved by reducing superfluous expenditures. Using analytics can assist in identifying opportunities to optimise operations and eliminate non-essential expenses from your business. There are three key ways that data analytic techniques can evaluate internal controls and ensure expenditure is in line with your organisation's policies.
Statistical analysis
Use advanced routines across cost data to identify potential outliers that aren't compliant with internal policies, such as missing discounts, duplicates, incorrect unit pricing, or unapproved suppliers.
Data visualisation
Utilise data visualisation to understand and analyse trends and behaviours of costs data. This will give an indication of how well internal controls are operating and if user behaviour is counter to the organisations ethics and policies. This can also be leveraged in future periods for benchmarking.
Cognitive detection
Use cognitive methods to understand if inappropriate items are being put forward as costs when they aren't allowable. By analysing descriptions of costs, you can detect keywords that suggest costs aren't appropriate or in line with internal policies. This could be employees claiming back items of a capital nature or personal expenses.
Preventing cash flow risks
It's important that businesses manage debtors and accurately assess accounts receivable on a monthly basis. Data analytics can be used to free up liquidity to maintain operational resilience, such as using prescriptive analytics to aid sensitivity analysis of daily forecasts, or to delay payments that are within pre-agreed payment terms.
Cash flow forecasting
Data analytics can analyse historical cash flow data, sales trends, and payment patterns to create accurate cash-flow forecasts. By understanding future cash flow projections, businesses can better plan their expenses, identify potential shortfalls, and take proactive measures to address liquidity challenges.
Receivables and payables analysis
By analysing accounts receivable and accounts payable data, data analytics can help identify late payments, bottlenecks in the payment process, and potential delinquencies. This enables businesses to prioritise collections efforts, negotiate payment terms, and manage vendor relationships effectively to optimise cash inflow and outflow.
Supply chain risk analytics
During times of economic uncertainty, the ability to cover the cost of goods can be challenging. Supply chains can involve complex arrangements reflecting the nature of commercial agreements in place. Therefore, it's important to negotiate with suppliers to make sure you're receiving favourable terms and that stock management is accurate.
Supplier risk assessment
Data analytics can assess supplier data, financial health, performance history, and market intelligence to evaluate supplier risks. By leveraging this information, businesses can proactively address underperforming suppliers, implement improvement initiatives, or consider alternative sourcing options to reduce supply chain risks. Analytics can be helpful to identify vulnerable suppliers and consider adjusting payment terms to ensure continuity from key suppliers. You can also use cognitive analytics to identify contracts with force majeure clauses to cover any SLA breaches, or identifying purchasing commitments that may now be onerous given the change in economic conditions.
Supplier and buyer analysis
Standardise primary characteristics that define the supplier and buyer population to identify trends and benchmarks for determining areas of risk, anomalous activity or lack of criteria required by commercial agreements, corporate policies, and local regulations.
Machine learning
Use artificial intelligence and machine learning to find patterns that can be indicators of risk, such as stock obsolescence, shrinkage, alternative suppliers, or unusual physical count variances. By considering various factors such as economic indicators, customer behaviour, and market dynamics, businesses can anticipate potential disruptions, adjust production and procurement strategies, and implement contingency plans to minimise risks.
Intelligent risk insights
Reducing payroll risks
Data analytics can be used to reduce payroll risks during a downturn by providing insights and facilitating effective risk management.
Fraud detection
Data analytics can analyse payroll data to identify patterns and anomalies that may indicate fraudulent activities, such as ghost employees, unauthorised changes to employee records, or suspicious payroll transactions. By monitoring and analysing payroll data, businesses can promptly detect and mitigate payroll fraud risks.
Compliance monitoring
Data analytics can help ensure compliance with labour laws, tax regulations, and internal policies related to payroll. By analysing payroll data, businesses can identify potential compliance issues, such as incorrect wage calculations or non-compliance with overtime rules. This enables timely corrective actions and minimises legal and financial risks.
Time and attendance analysis
Data analytics can analyse time and attendance data to identify discrepancies, such as excessive overtime or unauthorised absences.
Error detection and resolution
Data analytics can identify errors or inconsistencies in payroll data, such as missing or incorrect entries, duplicate records, or calculation errors. By proactively detecting and resolving these issues, businesses can ensure accurate payroll processing and reduce the risk of financial penalties or employee dissatisfaction.
Stay ahead of the curve
Data analytics can provide valuable insights, enabling you to make data-driven decisions, optimise operations, and adapt to changing market conditions in a downturn. Ensure you're optimising your data to not only prevent risks, but add commercial benefits.
For more insight and guidance, get in touch with Alex Hunt.
Get the latest insights, events and guidance, straight to your inbox.