-
Governance advisory
We guide boards and management teams in frameworks, team processes and leadership dynamics to deliver sustainable value.
-
Financial services advisory
Get market-driven expertise to achieve your goals in banking, insurance, capital markets, and investment management.
-
Business risk services
Our market-driven expertise helps firms keep growing and manage risk in an evolving regulatory landscape.
-
Risk
Meet risks with confidence and transform your business – we support you to manage risk and deliver on your goals.
-
Economic consulting
Bespoke guidance grounded in complex economic theory and practical sector insight to help you make the right decisions.
-
Government and public sector
Experience and expertise in delivering quality public sector advisory and audits.
-
Business consulting
Partnering with you to deliver sustainable business change that helps you realise your ambitions.
-
Transaction advisory services
Whether buying or selling, we help you get the deal done with our comprehensive range of transaction advisory services.
-
Financial accounting advisory services (FAAS)
Our FAAS team can support your finance function with the flexible resource they need to get results.
-
Corporate finance advisory
Building a business is never easy. We help you maximise the value of your business and find the right option.
-
Valuations
Help to understand or support the valuation of a business or asset.
-
Insolvency and global asset recovery
We provide asset tracing and seamless cross-border global recovery for clients.
-
Forensic and investigation services
Market-driven expertise in investigations, dispute resolution and digital forensics.
-
Restructuring
Our restructuring team help lenders, investors and management navigate contingency plans, restructuring and insolvency.
-
Transformation consulting
Is business transformation a priority for your organisation? Our expert insight and guidance can help you achieve it.
-
Pensions assurance
A tailored service that responds to evolving risks and regulations.
-
Accounting services
Optimise your growth with expert accounting services. Contact us today.
-
Royalty and intellectual property (IP) audits
Enhance IP asset protection with our royalty and IP audit services. Expertise in licensing, revenue detection, and compliance improvements.

-
Business consulting
Partnering with you to deliver sustainable business change that helps you realise your ambitions.
-
Corporate Simplification
Release value, reduce compliance complexity, and improve tax efficiency by streamlining your group structure.
-
Economic consulting
Bespoke guidance grounded in complex economic theory and practical sector insight to help you make the right decisions.
-
Financial accounting advisory services (FAAS)
Our FAAS team can support your finance function with the flexible resource they need to get results.
-
Governance advisory
We guide boards and management teams in frameworks, team processes and leadership dynamics to deliver sustainable value.
-
International
Unlock global opportunities with our local expertise and worldwide reach.
-
People advisory
Driving business performance through people strategy and culture.
-
Strategy Group
Successful business strategy is rooted in a clear understanding of the market, customer segmentation and how purchase decisions vary.
-
Respond: Data breach, incident response and computer forensics
Are you prepared for a cyber failure? We can help you avoid data breaches and offer support if the worst happens.
-
Comply: Cyber security regulation and compliance
Cyber security regulation and compliance is constantly evolving. Our team can support you through the digital landscape.
-
Protect: Cyber security strategy, testing and risk assessment
Cyber security threats are constantly evolving. We’ll work with you to develop and test robust people, process and technology defences to protect your data and information assets.
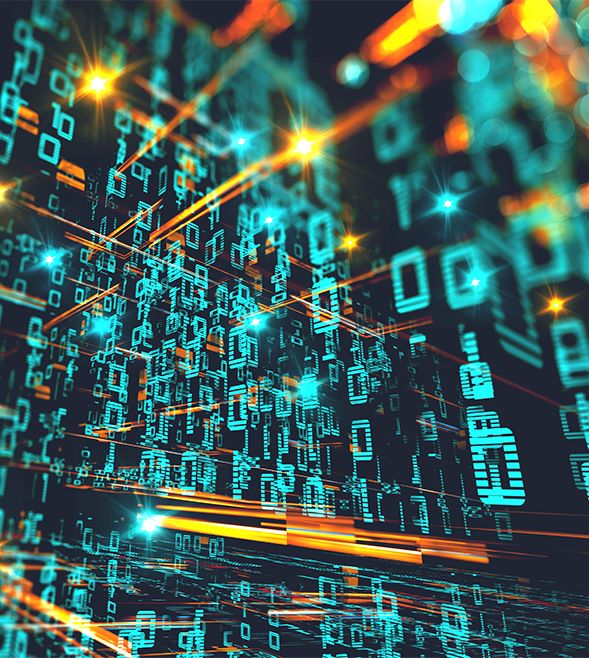
-
Corporate finance advisory
Building a business is never easy. We help you maximise the value of your business and find the right option.
-
Debt advisory
Working with borrowers and private equity financial sponsors on raising and refinancing debt. We can help you find the right lender and type of debt products.
-
Financial accounting advisory services (FAAS)
Our FAAS team can support your finance function with the flexible resource they need to get results.
-
Financial modelling services
Financial modelling that helps you wrestle with your most pressing business decisions.
-
Operational deal services
Enabling transaction goals through due diligence, integration, separation, and other complex change.
-
Our credentials
Search our transactions to see our experience in your sector and explore the deals advisory services we've delivered.
-
Transaction advisory services
Whether buying or selling, we help you get the deal done with our comprehensive range of transaction advisory services.
-
Valuations
Help to understand or support the valuation of a business or asset.
-
The ESG agenda
Shape your ESG agenda by identifying the right metrics, sustainable development and potential business value impact.
-
ESG driven business transition
Whatever your ESG strategy, we can support your organisation as it evolves while maximising efficiency and profitability.
-
ESG programme and change management
Do you have the right capabilities to drive the delivery of your ESG strategy to realise your targets?
-
ESG risk management
You must protect, comply, understand and influence to successfully manage the risk involved with ESG issues. We can help.
-
ESG strategy, risk and opportunity identification
We can help you clearly define your ESG Strategy, with the risks and opportunities identified and managed.
-
Create value through effective ESG communication
Building trust and engagement with your stakeholders on your ESG strategy.
-
ESG metrics, targets and disclosures
The pressure to report your ESG progress is growing. Do your targets measure up?
-
ESG governance, leadership and culture framework
Make the most of ESG opportunities by effectively embedding your strategy across your organisation.
-
ESG and non-financial assurance
Support your board to be confident in supplying robust information that withstands scrutiny.
-
Transition planning to net zero
Supporting your organisation in the transition to net zero.
-
Actuarial and insurance consulting
We consult extensively to the life insurance, general insurance, health insurance and pensions sectors.
-
Business risk services
Our market-driven expertise helps firms keep growing and manage risk in an evolving regulatory landscape.
-
Financial crime
Helping you fight financial crime in a constantly changing environment
-
Financial services business consulting
Leverage our diverse capabilities to manage challenges and take opportunities: from assurance to transformation
-
Financial services tax
Helping financial services firms navigate the global financial services and funds tax landscape.
-
Regulatory and compliance
Providing an exceptional level of regulatory and compliance to firms across the financial services industry.
-
Corporate intelligence
Corporate intelligence often involves cross-border complexities. Our experienced team can offer support.
-
Litigation support
Industry-wide litigation support and investigation services for lawyers and law firms.
-
Disputes advisory
Advising on quantum, accounting and financial issues in commercial disputes.
-
Forensic investigations and special situations
Do you need clarity in an uncertain situation? If you're accused of wrongdoing we can help you get the facts right.
-
Forensic data analytics
Our forensic data analytics team are helping businesses sift the truth from their data. See how we can help your firm.
-
Monitoring trustee and competition services
Monitoring trustee services to competition, financial and regulatory bodies.
-
Financial crime
Supporting your fight against financial crime in an ever-changing environment
-
Whistleblowing and investigation support
Whistleblowing frameworks provide many benefits – find out how to build trust and manage risks in a confidential, cost-effective, robust way.
-
Public sector advisory
To deliver excellent public services, local and central government need specialist support.
-
Public sector consulting
Helping public sector organisations maintain oversight of services and understand what's happening on the ground.
-
Public sector audit and assurance
As a leading UK auditor, we have unparalleled insights into the risks, challenges and opportunities that you face.
-
Competition damages and class actions
Helping you recover damages owed from anti-competitive practices.
-
Contentious estates and family disputes
We manage complex and sensitive disputes through to resolution.
-
Digital Asset Recovery
Get guidance and technical expertise on digital finance and cryptoasset recovery from our dedicated crypto hub.
-
Grant Thornton Offshore
Grant Thornton Offshore is our one-stop global solution for insolvency, asset recovery, restructuring and forensics services.
-
Insolvency Act Portal
Case information and published reports on insolvency cases handled by Grant Thornton UK LLP.
-
Litigation support
Industry-wide litigation support and investigation services for lawyers and law firms.
-
Personal insolvency
We can support you to maximise personal insolvency recovery and seek appropriate debt relief.
-
South Asia business group
We help Indian companies expand into the UK and invest globally. We also help UK companies invest and operate in India.
-
US business group
Optimise your trans-Atlantic operations with local knowledge and global reach.
-
Japan business group
Bridging the commercial and cultural divide and supporting your ambitions across Japan and the UK.
-
Africa business group
Connecting you to the right local teams in the UK, Africa, and the relevant offshore centres.
-
China-Britain business group
Supporting your operations across the China – UK economic corridor.
-
Asset based lending advisory
Helping lenders, their clients and other stakeholders navigate the complexities of ABL.
-
Contingency planning and administrations
In times of financial difficulty, it is vital that directors explore all the options that are available to them, including having a robust ‘Plan B’.
-
Corporate restructuring
Corporate restructuring can be a difficult time. Let our team make the process simple and as stress-free as possible.
-
Creditor and lender advisory
Whether you're a creditor or lender, complex restructurings depend on pragmatic commercial advice
-
Debt advisory
Our debt advisory team can find the right lender to help you in restructuring. Find out how our experts can support you.
-
Financial services restructuring and insolvency
Financial services restructuring and insolvency is a competitive marketplace. Our team can help you navigate this space.
-
Pensions advisory services
DB pension-schemes need a balanced approach that manages risk for trustees and sponsors in an uncertain economy.
-
Restructuring and insolvency tax
Tax will often be crucial in a plan to restructure a distressed business. Our team can guide you through the process.
-
Restructuring Plans
Market leading experience in advising companies and creditors in Restructuring Plan processes.
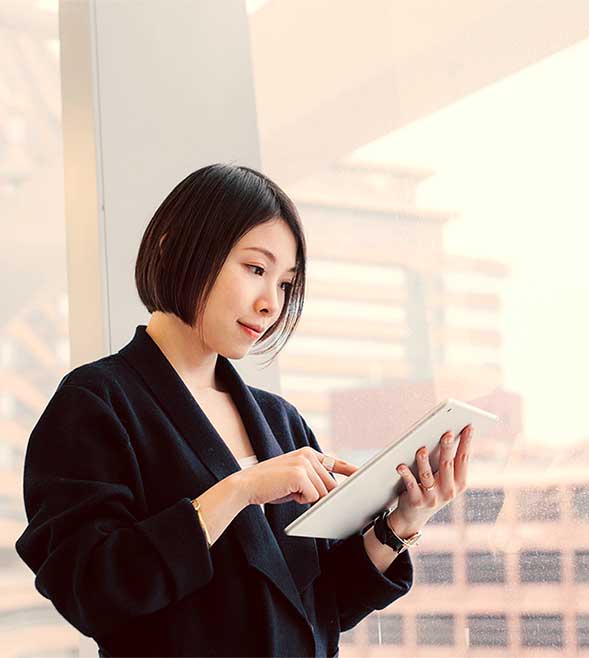
-
Artificial intelligence
Our approach to the design, development, and deployment of AI systems can assist with your compliance and regulation.
-
Controls advisory
Build a robust internal control environment in a changing world.
-
Data assurance and analytics
Enhancing your data processes, tools and internal capabilities to help you make decisions on managing risk and controls.
-
Enterprise risk management
Understand and embrace enterprise risk management – we help you develop and connect risk thinking to your objectives.
-
Internal audit services
Internal audit services that deliver the value and impact they should.
-
Managing risk and realising ESG opportunities
Assess and assure risk and opportunities across ESG with an expert, commercial and pragmatic approach.
-
Project, programme, and portfolio assurance
Successfully delivering projects and programmes include preparing for the wider impact on your business.
-
Service organisation controls report
Independent assurance provides confidence to your customers in relation to your services and control environment.
-
Supplier and contract assurance
Clarity around key supplier relationships: focusing on risk, cost, and operational performance.
-
Technology risk services
IT internal audits and technology risk assurance projects that help you manage your technology risks effectively.
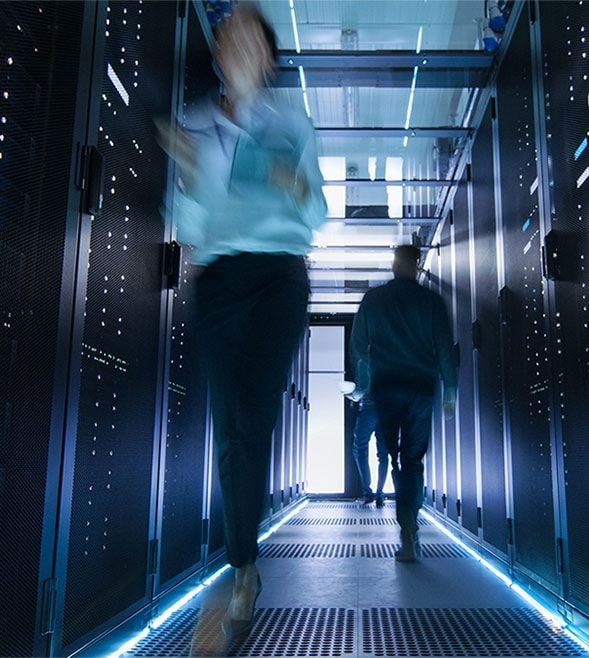
-
Capital allowances (tax depreciation)
Advisory and tools to help you realise opportunities in capital allowances.
-
Corporate tax
Helping companies manage corporate tax affairs: delivering actionable guidance to take opportunities and mitigate risk.
-
Employer solutions
We will help you deliver value through your employees, offering pragmatic employer solutions to increasing costs.
-
Financial services tax
Helping financial services firms navigate the global financial services and funds tax landscape.
-
Indirect tax
Businesses face complex ever changing VAT regimes, guidance and legislation. We can help you navigate these challenges.
-
International tax
Real-world international tax advice to help you navigate a changing global tax landscape.
-
Our approach to tax
We advise clients on tax law in the UK and, where relevant, other jurisdictions.
-
Private tax
Tax experts for entrepreneurs, families and private business. For now and the long term.
-
Real estate tax
Stay ahead of real estate tax changes with holistic, tax-efficient solutions.
-
Research and development tax incentives
We can help you prepare optimised and robust research and development tax claims.
-
Tax dispute resolution
We make it simple to stay compliant and avoid HMRC tax disputes
-
Tax risk management
We work with you to develop effective tax risk management strategies.
-
Skills and training
Get the right support to deliver corporate and vocational training that leads the way in an expanding market.
-
Private education
Insight and guidance for all businesses in the private education sector: from early years to higher education and edtech.
-
Facilities management and property services
Get insight and strategic support to take opportunities that protect resilience and drive UK and international growth.
-
Recruitment
Helping recruitment companies take opportunities to achieve their goals in a market where talent and skills are key.
-
Food and beverage (F&B)
We can help you find the right ingredients for growth in your food and beverage business.
-
Travel, tourism and leisure
Tap into our range of support for travel, tourism and leisure businesses in this period of challenge and change.
-
Retail, e-commerce and consumer products
With multiple challenges and opportunities in the fast-evolving retail sector, make sure you are ready for them.
-
Banking
Our expertise and insight can help you respond positively to long term and emerging issues in the banking sector.
-
Capital markets
2020 is a demanding year for capital markets. Working with you, we're architecting the future of the sector.
-
Insurance
Our experienced expert team brings you technical expertise and insight to guide you through insurance sector challenges.
-
Investment management
Embracing innovation and shaping business models for long-term success.
-
Pensions
Pension provision is an essential issue for employers, and the role of the trustee is becoming increasingly challenging.
-
Payments advisory and assurance
Payment service providers need to respond to rapidly evolving technical innovations and increased regulatory scrutiny.
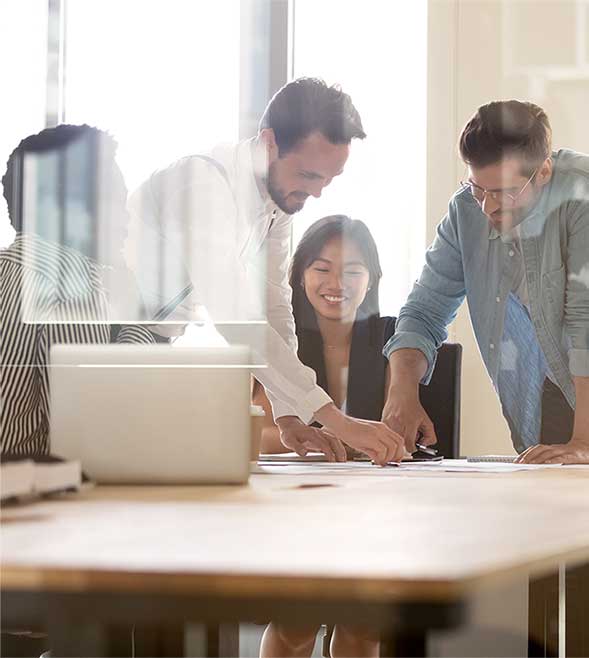
-
Central and devolved government
Helping central and devolved governments deliver change to improve our communities and grow our economies.
-
Infrastructure and transport
Delivering a successful transport or infrastructure project will require you to balance an often complex set of strategic issues.
-
Local government
Helping local government leverage technical and strategic expertise deliver their agendas and improve public services.
-
Regeneration development and housing
We provide commercial and strategic advice to assist your decision making in pursuing your objectives.
-
Health and social care
Sharing insight and knowledge to deliver transformation and improvement to health and social care services.
-
Charities
Supporting you to achieve positive change in the UK charity sector.
-
Education and skills
The education sector has rarely faced more risk or more opportunity to transform. You need to plan for the future.
-
Social housing
We are committed to helping change social housing for the better, and can help you make the most of every opportunity.

-
Technology
We work with dynamic technology companies of all sizes to help them succeed and grow internationally.
-
Telecommunications
Take all opportunities to realise your goals in telecommunications: from business refresh to international expansion.
-
Media
Media companies must stay agile to thrive in today’s highly competitive market – we’re here to support your ambitions.
-
Career development
The support you need will vary throughout your career, here are just some of the ways we'll support you to you excel with us.
-
How we work
Our approach to how we work, ensures that when we are making choices about how, where or when we work, we have the support and tools we need.
-
Reward and benefits
We are committed to building a culture where our people have access to the necessary benefits to help promote a healthy lifestyle and thrive.
-
Inclusion and diversity
Included and valued for your difference is how everyone should feel at work. Not just because it’s right, but because we’re all at our best when we’re able to be ourselves.
-
ESG: environment and community impact
Our ESG framework enables responsible, sustainable, and ethical operations. We prioritise the environment, our broader societal impact, and our firm's governance to protect the planet, foster inclusivity and wellbeing, support our communities, and bolster our firm's resilience.
-
What we do
It’s an exciting time to be joining Grant Thornton, especially as a trainee at the start of your career. Learn about our teams and the work we do.
-
Life as a trainee
Everything you need to know about life as a trainee, from the experiences you'll get to the skills you need.
-
Employability hub
Our employability hub is designed to help you feel prepared for the application process, and guide you through the decisions you will need to make throughout.
-
Our programmes
Whether you are looking to join us straight from school or with a degree, or even looking for some work experience, we have a programme that is right for you.
-
Parents, carers and teachers hub
Access information and support to help inform career discussions with your young people.
-
FAQs
We hope you can find all the information you need on our website, but to help we've collated a few of the questions we hear quite frequently when speaking to candidates.
-
Advisory
Our advisory practice provides organisations with the advice and solutions they need to unlock sustainable growth and navigate complex risks and challenges.
-
Audit
Every day our audit teams help people in businesses and communities to do what is right and achieve their goals.
-
Tax
The tax landscape is evolving, and our clients need us more than ever to navigate the complexities with them.
-
Central Client Service
From finance and information systems, to marketing and people teams we have a diverse range of teams supporting our business.
-
Our recruitment process
We strive to ensure our interview process is barrier free and sets you up for success, as well as being wholly inclusive and robust. Learn about our process here.
-
International hiring
Our international talent is an important part of our firm, joining us you will be joining a growing community of international hires.
-
Armed forces
As an Armed Forces Friendly organisation we are proud to support members of the Armed Forces Community as they develop their career outside of the Armed Forces.
Used effectively, models are a useful tool to manage credit, operational and market risk, and more. But they rely on good quality data, and regulators are increasingly concerned about the level of assurance banks can provide.
New principles for model risk management has been finalised last week, but at this stage the policy will only be applicable to organisations with internal model (IM) approval to calculate regulatory capital requirements. Due to the outstanding final definition of ‘Simpler-regime Firms’ under the Strong & Simple Framework, the PRA will provide an update on the approach for all other firms, including ‘Simpler-regime Firms’, at a future date, once the definition of a ‘Simpler-regime Firm’ has been finalised.
The policy will take effect 12 months after publication on Friday 17 May 2024. Organisations that first receive permission to use an internal model to calculate regulatory capital after this date will have 12 months from the grant of that permission to comply with the expectations in SS1/23.
By the implementation date, firms must conduct an initial self-assessment to map their readiness to adopt the new principles, identifying areas they need to build or enhance their MRM capabilities and framework. Self-assessments should be updated at least annually thereafter, with findings and remediations plans from the self- assessment documented and shared with the board.
The five principles
Taking a closer look at SS1/23, the PRA isn’t suggesting a whole new approach to model risk management. The key principles cover the following five areas. In addition to the increased scope of models and consequent increased validation pipeline, the PRA is introducing principles with a focus on underlying data quality. It is formalising expectations around data sources and lineage, and how that data is used in modelling, across every stage of the model life cycle.
Principle 1 – Model identification and model risk classification
Firms should have an established definition of a model that sets the scope for MRM, a model inventory, and a risk-based tiering approach to categorise models to help identify and manage model risk. Specifically, when assessing a model's complexity, you need to consider the nature and quality of the input data, the use of alternative and unstructured data, and the potential for designer or data bias to be present.
Principle 2 – Governance
Firms should have strong governance oversight with a board that promotes an MRM culture from the top through setting a clear model risk appetite. The board approves the MRM policy and appoints an accountable individuals responsible for implementing a sound MRM framework. This includes adequate systems and infrastructure to maintain data and system integrity. Data quality procedures and standards should set clear roles and responsibilities for managing quality of data used for model development, and the rules and standards for data quality, accuracy and relevance.
Principle 3 – Model development, implementation and use
Firms should have a robust model development process with standards for model design and implementation, model selection, and model performance measurement. Documentation should describe the use of data, data sources, data proxies, and the results of data quality, accuracy and relevance tests. This includes documenting any data adjustments, which are subject to validation; and details of any interconnected sources, alternative and unstructured data should be recorded in the model inventory. You should also ensure no inappropriate bias is used to develop the model, and that data is compliant with data privacy and other data regulations.
Principle 4 – Independent model validation
Firms should have a validation process that provides ongoing, independent and effective challenge to model development and use. The validation function should provide an objective, unbiased and critical opinion on the relevance and completeness of the development data with respect to the underlying portfolios, products, assets or customer base the model will be used for. It also shares the responsibility for the verification of model processes and system implementation which includes verification of the representativeness of internal or external data used as model inputs and compliance with internal data quality control and reliability standards.
Principle 5 – Model risk mitigants
Firms should have established policies and procedures for mitigating model risk when models are underperforming, and procedures for the independent review of post-model adjustments (PMA). All PMAs should be subject to an independent review, including assessing inputs to ensure data integrity and to ensure it remains representative of the underlying portfolio.
Reshaping model risk processes to new regulation
Most firms will already have processes in place in these five areas; they just need to map and reshape them to meet new regulatory expectations. To date, most financial institutions have based their data governance approaches on BCBS 239, which was introduced in 2013 to improve risk data aggregation capabilities.
Although the BCBS 239 principles were initially aimed at global systemically important banks (G-SIBs), the principles have become a standard across the banking industry, as local supervisors follow the Basel Committee on Banking Supervision (BCBS) recommendations and apply the principles to domestic systemically important banks (D-SIBs). BCBS 239 is therefore a good place to start when mapping readiness for the PRA’s new principles regarding data.
Creating synergies with BCBS 239
Starting with your practices for BCBS 239, it’s fairly straightforward to see the overlap with the PRA’s new requirements and to find the areas where you’ll need to undertake additional work.
Governance, data architecture and IT infrastructure
Under BCBS 239, firms need strong governance arrangements with defined roles and responsibilities, open forums and clear escalation channels to improve accountability and reporting. These elements are supported by data taxonomies to improve communication and standardise terminology, and effective policies and procedures on data management.
How this maps to SS1/23:
Principle 2.2
Looking at the senior management function (SMF) accountability for model risk management framework, including resourcing, adequate systems and infrastructure to ensure data and system integrity; also effective controls and testing of model outputs.
Principle 4.1
Calling for independent validation for an objective, unbiased and critical opinion on the accuracy, relevance and completeness of the development data, output and reports.
Principle 4.2
Requiring robust independent review to critically analyse the quality and extent of model development evidence, including the relevance and completeness of the data used to develop the model.
Risk data aggregation capabilities
Under BCBS 239, data aggregation should be largely automated to support accurate and reliable risk data for reporting purposes, including during stressed conditions. Data must be complete, timely and available on an ad hoc basis. Key tools such as a data dictionary, data quality controls and flexible data aggregation capabilities and process documentation support this outlook.
How this maps to SS1/23:
Principle 2.3
Covering policies and procedures for model tiering, including data sources, quality management procedures (including rules and standards, accuracy and relevance); also specific risk controls and criteria for use of alternative or unstructured data, or information sources. For data intensive models, firms must consider responsibilities for managing data quality.
Principle 3.5
Targeting model development documentation, including: the use of data, a description of the data sources, any data proxies, and the results of data quality, accuracy and relevance tests.
Principle 4.3
Looking at process verification around model inputs from internal or external data, to make sure they’re representative of the underlying portfolios, products, assets or customer base the model will be used for.
Risk reporting practices
To improve risk reporting practices, BCBS 239 focuses on improving accuracy over aggregated risk data, comprehensiveness, clarity and frequency. This depends on having effective data quality dashboards, robust risk management reporting practices, good issue management and recognition of any limitations of material gaps.
How this maps to SS1/23:
Principle 2.6
Looking at externally developed models, third-party and vendor products to verify the relevance of vendor supplied data and their assumptions. This includes models developed by parent groups, and subsidiaries should check the data is relevant for the intended model.
Principle 3.2
Covering the use of data, including checking that it’s suitable for the intended use case, consistent with the methodology, and representative of underlying portfolios, products, assets, or customer base. Model development processes should assess potential data bias and ensure regulatory compliance. Firms should document data adjustments or use of proxies, which are subject to validation. The model inventory should include any interconnected data sources, and record alternative or unstructured data, which should be reflected in the model’s tier classification.
Preparing your SS1/23 readiness assessment
In the short term you must review your existing data infrastructure, related systems and processes against the SS1/23 principles to complete the PRA’s upcoming self-assessment requirement. This must include key elements of the model life cycle, model development and validation of models, in addition to data infrastructure and related systems. If you find any shortcomings, you need to put remediation plans in place to bring your model risk data quality processes in line with regulatory expectations.
Typical stumbling blocks include manual data collection, and incomplete or inconsistent data. So, it’s essential to improve data aggregation and management capabilities at every stage of the model life cycle: from collection and storage to analysis and reporting.
With the final rules landed, it’s important to think about how to leverage your existing investments in data to enhance the quality of data sources and systems for models. This will boost confidence in your model reliability and support decision-making processes.
For more information and advice, contact Vivian Lagan.