-
Governance advisory
We guide boards and management teams in frameworks, team processes and leadership dynamics to deliver sustainable value.
-
Financial services advisory
Get market-driven expertise to achieve your goals in banking, insurance, capital markets, and investment management.
-
Business risk services
Our market-driven expertise helps firms keep growing and manage risk in an evolving regulatory landscape.
-
Risk
Meet risks with confidence and transform your business – we support you to manage risk and deliver on your goals.
-
Economic consulting
Bespoke guidance grounded in complex economic theory and practical sector insight to help you make the right decisions.
-
Government and public sector
Experience and expertise in delivering quality public sector advisory and audits.
-
Business consulting
Partnering with you to deliver sustainable business change that helps you realise your ambitions.
-
Transaction advisory services
Whether buying or selling, we help you get the deal done with our comprehensive range of transaction advisory services.
-
Financial accounting advisory services (FAAS)
Our FAAS team can support your finance function with the flexible resource they need to get results.
-
Corporate finance advisory
Building a business is never easy. We help you maximise the value of your business and find the right option.
-
Valuations
Help to understand or support the valuation of a business or asset.
-
Insolvency and global asset recovery
We provide asset tracing and seamless cross-border global recovery for clients.
-
Forensic and investigation services
Market-driven expertise in investigations, dispute resolution and digital forensics.
-
Restructuring
Our restructuring team help lenders, investors and management navigate contingency plans, restructuring and insolvency.
-
Transformation consulting
Is business transformation a priority for your organisation? Our expert insight and guidance can help you achieve it.
-
Pensions assurance
A tailored service that responds to evolving risks and regulations.
-
Pensions assurance
A tailored service that responds to evolving risks and regulations.
-
Accounting services
Optimise your growth with expert accounting services. Contact us today.
-
Royalty and intellectual property (IP) audits
Enhance IP asset protection with our royalty and IP audit services. Expertise in licensing, revenue detection, and compliance improvements.
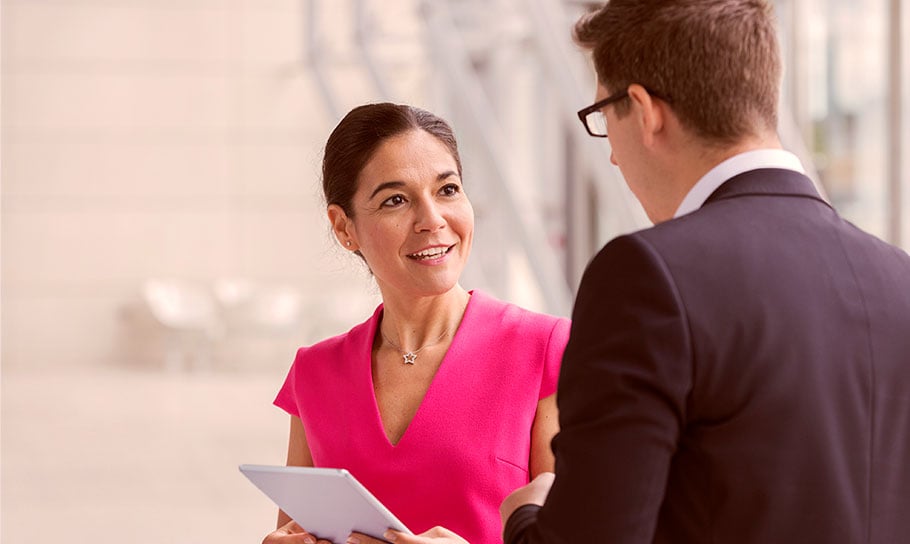
-
Business consulting
Partnering with you to deliver sustainable business change that helps you realise your ambitions.
-
Corporate Simplification
Release value, reduce compliance complexity, and improve tax efficiency by streamlining your group structure.
-
Economic consulting
Bespoke guidance grounded in complex economic theory and practical sector insight to help you make the right decisions.
-
Governance advisory
We guide boards and management teams in frameworks, team processes and leadership dynamics to deliver sustainable value.
-
International
Unlock global opportunities with our local expertise and worldwide reach.
-
People advisory
Driving business performance through people strategy and culture.
-
Strategy Group
Successful business strategy is rooted in a clear understanding of the market, customer segmentation and how purchase decisions vary.
-
Respond: Data breach, incident response and computer forensics
Are you prepared for a cyber failure? We can help you avoid data breaches and offer support if the worst happens.
-
Comply: Cyber security regulation and compliance
Cyber security regulation and compliance is constantly evolving. Our team can support you through the digital landscape.
-
Protect: Cyber security strategy, testing and risk assessment
Cyber security threats are constantly evolving. We’ll work with you to develop and test robust people, process and technology defences to protect your data and information assets.
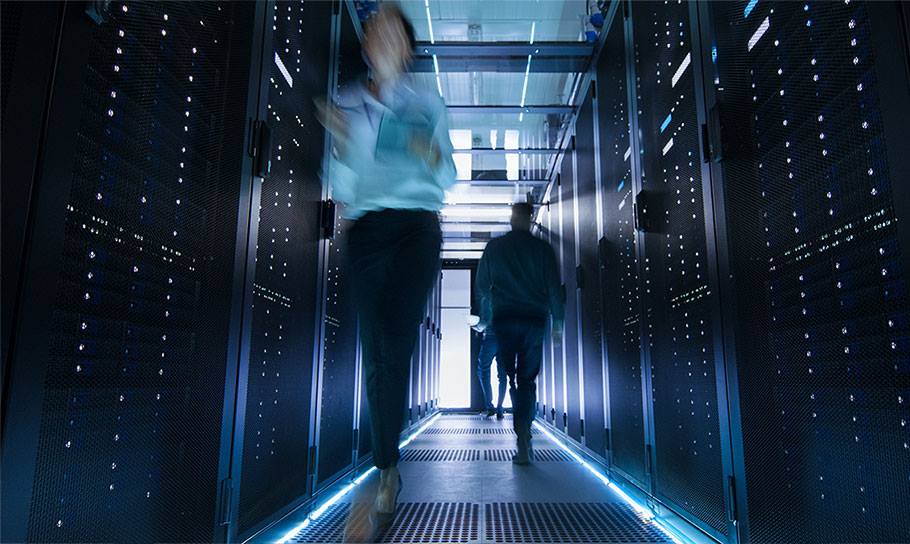
-
Corporate finance advisory
Building a business is never easy. We help you maximise the value of your business and find the right option.
-
Debt advisory
Working with borrowers and private equity financial sponsors on raising and refinancing debt. We can help you find the right lender and type of debt products.
-
Financial accounting advisory services (FAAS)
Our FAAS team can support your finance function with the flexible resource they need to get results.
-
Financial modelling services
Financial modelling that helps you wrestle with your most pressing business decisions.
-
Operational deal services
Enabling transaction goals through due diligence, integration, separation, and other complex change.
-
Our credentials
Search our transactions to see our experience in your sector and explore the deals advisory services we've delivered.
-
Transaction advisory services
Whether buying or selling, we help you get the deal done with our comprehensive range of transaction advisory services.
-
Valuations
Help to understand or support the valuation of a business or asset.
-
The ESG agenda
Shape your ESG agenda by identifying the right metrics, sustainable development and potential business value impact.
-
ESG driven business transition
Whatever your ESG strategy, we can support your organisation as it evolves while maximising efficiency and profitability.
-
ESG programme and change management
Do you have the right capabilities to drive the delivery of your ESG strategy to realise your targets?
-
ESG risk management
You must protect, comply, understand and influence to successfully manage the risk involved with ESG issues. We can help.
-
ESG strategy, risk and opportunity identification
We can help you clearly define your ESG Strategy, with the risks and opportunities identified and managed.
-
Create value through effective ESG communication
Building trust and engagement with your stakeholders on your ESG strategy.
-
ESG metrics, targets and disclosures
The pressure to report your ESG progress is growing. Do your targets measure up?
-
ESG governance, leadership and culture framework
Make the most of ESG opportunities by effectively embedding your strategy across your organisation.
-
ESG and non-financial assurance
Support your board to be confident in supplying robust information that withstands scrutiny.
-
Actuarial and insurance consulting
We consult extensively to the life insurance, general insurance, health insurance and pensions sectors.
-
Business risk services
Our market-driven expertise helps firms keep growing and manage risk in an evolving regulatory landscape.
-
Financial crime
Helping you fight financial crime in a constantly changing environment
-
Financial services business consulting
Leverage our diverse capabilities to manage challenges and take opportunities: from assurance to transformation
-
Financial services tax
Helping financial services firms navigate the global financial services and funds tax landscape.
-
Regulatory and compliance
Providing an exceptional level of regulatory and compliance to firms across the financial services industry.
-
Corporate intelligence
Corporate intelligence often involves cross-border complexities. Our experienced team can offer support.
-
Litigation support
Industry-wide litigation support and investigation services for lawyers and law firms.
-
Disputes advisory
Advising on quantum, accounting and financial issues in commercial disputes.
-
Forensic investigations and special situations
Do you need clarity in an uncertain situation? If you're accused of wrongdoing we can help you get the facts right.
-
Forensic data analytics
Our forensic data analytics team are helping businesses sift the truth from their data. See how we can help your firm.
-
Monitoring trustee and competition services
Monitoring trustee services to competition, financial and regulatory bodies.
-
Financial crime
Supporting your fight against financial crime in an ever-changing environment
-
Public sector advisory
To deliver excellent public services, local and central government need specialist support.
-
Public sector consulting
Helping public sector organisations maintain oversight of services and understand what's happening on the ground.
-
Public sector audit and assurance
As a leading UK auditor, we have unparalleled insights into the risks, challenges and opportunities that you face.
-
Contentious estates and family disputes
We manage complex and sensitive disputes through to resolution.
-
Digital Asset Recovery
Get guidance and technical expertise on digital finance and cryptoasset recovery from our dedicated crypto hub.
-
Grant Thornton Offshore
Grant Thornton Offshore is our one-stop global solution for insolvency, asset recovery, restructuring and forensics services.
-
Insolvency Act Portal
Case information and published reports on insolvency cases handled by Grant Thornton UK LLP.
-
Litigation support
Industry-wide litigation support and investigation services for lawyers and law firms.
-
Personal insolvency
We can support you to maximise personal insolvency recovery and seek appropriate debt relief.
-
South Asia business group
Supporting your growth in the UK-India economic corridor and beyond.
-
US business group
Optimise your trans-Atlantic operations with local knowledge and global reach.
-
Japan business group
Bridging the commercial and cultural divide and supporting your ambitions across Japan and the UK.
-
Africa business group
Connecting you to the right local teams in the UK, Africa, and the relevant offshore centres.
-
China-Britain business group
Supporting your operations across the China – UK economic corridor.
-
Asset based lending advisory
Helping lenders, their clients and other stakeholders navigate the complexities of ABL.
-
Contingency planning and administrations
In times of financial difficulty, it is vital that directors explore all the options that are available to them, including having a robust ‘Plan B’.
-
Corporate restructuring
Corporate restructuring can be a difficult time. Let our team make the process simple and as stress-free as possible.
-
Creditor and lender advisory
Whether you're a creditor or lender, complex restructurings depend on pragmatic commercial advice
-
Debt advisory
Our debt advisory team can find the right lender to help you in restructuring. Find out how our experts can support you.
-
Financial services restructuring and insolvency
Financial services restructuring and insolvency is a competitive marketplace. Our team can help you navigate this space.
-
Pensions advisory services
DB pension-schemes need a balanced approach that manages risk for trustees and sponsors in an uncertain economy.
-
Restructuring and insolvency tax
Tax will often be crucial in a plan to restructure a distressed business. Our team can guide you through the process.
-
Restructuring Plans
Market leading experience in advising companies and creditors in Restructuring Plan processes.
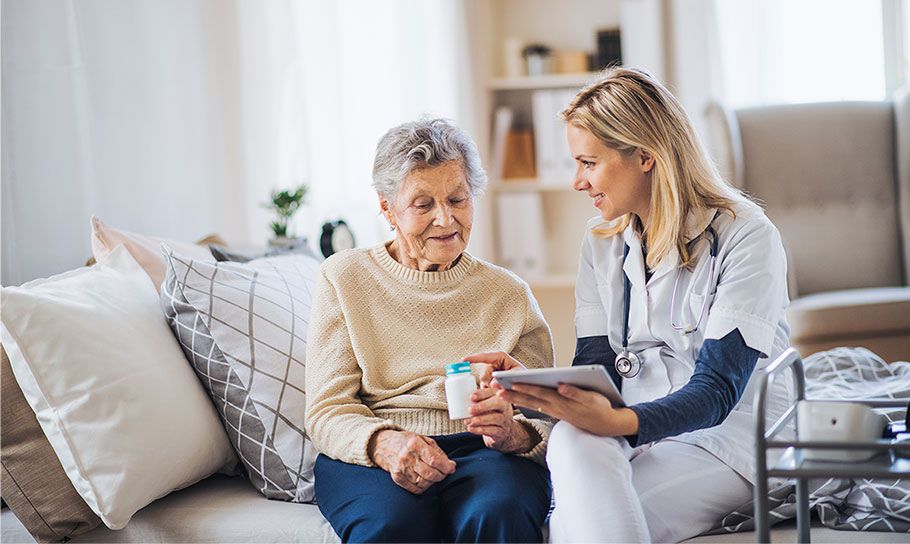
-
Controls advisory
Build a robust internal control environment in a changing world.
-
Data assurance and analytics
Enhancing your data processes, tools and internal capabilities to help you make decisions on managing risk and controls.
-
Enterprise risk management
Understand and embrace enterprise risk management – we help you develop and connect risk thinking to your objectives.
-
Internal audit services
Internal audit services that deliver the value and impact they should.
-
Managing risk and realising ESG opportunities
Assess and assure risk and opportunities across ESG with an expert, commercial and pragmatic approach.
-
Project, programme, and portfolio assurance
Successfully delivering projects and programmes include preparing for the wider impact on your business.
-
Service organisation controls report
Independent assurance provides confidence to your customers in relation to your services and control environment.
-
Supplier and contract assurance
Clarity around key supplier relationships: focusing on risk, cost, and operational performance.
-
Technology risk services
IT internal audits and technology risk assurance projects that help you manage your technology risks effectively.
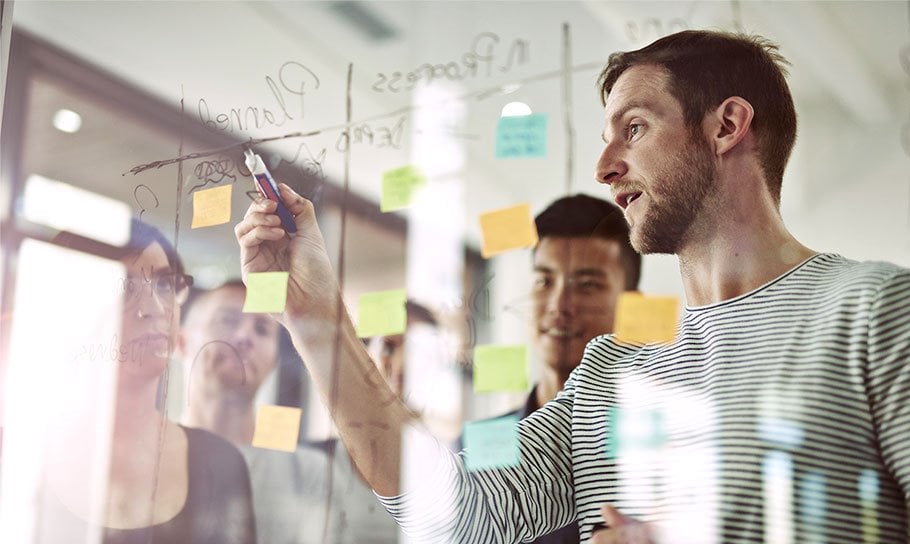
-
Capital allowances (tax depreciation)
Advisory and tools to help you realise opportunities in capital allowances.
-
Corporate tax
Helping companies manage corporate tax affairs: delivering actionable guidance to take opportunities and mitigate risk.
-
Employer solutions
We will help you deliver value through your employees, offering pragmatic employer solutions to increasing costs.
-
Indirect tax
Businesses face complex ever changing VAT regimes, guidance and legislation. We can help you navigate these challenges.
-
International tax
Real-world international tax advice to help you navigate a changing global tax landscape.
-
Our approach to tax
We advise clients on tax law in the UK and, where relevant, other jurisdictions.
-
Private tax
Tax experts for entrepreneurs, families and private business. For now and the long term.
-
Real estate tax
Stay ahead of real estate tax changes with holistic, tax-efficient solutions.
-
Research and development tax incentives
We can help you prepare optimised and robust research and development tax claims.
-
Tax dispute resolution
We make it simple to stay compliant and avoid HMRC tax disputes
-
Tax risk management
We work with you to develop effective tax risk management strategies.
-
Skills and training
Get the right support to deliver corporate and vocational training that leads the way in an expanding market.
-
Private education
Insight and guidance for all businesses in the private education sector: from early years to higher education and edtech.
-
Facilities management and property services
Get insight and strategic support to take opportunities that protect resilience and drive UK and international growth.
-
Recruitment
Helping recruitment companies take opportunities to achieve their goals in a market where talent and skills are key.
-
Food and beverage (F&B)
We can help you find the right ingredients for growth in your food and beverage business.
-
Travel, tourism and leisure
Tap into our range of support for travel, tourism and leisure businesses in this period of challenge and change.
-
Retail, e-commerce and consumer products
With multiple challenges and opportunities in the fast-evolving retail sector, make sure you are ready for them.
-
Banking
Our expertise and insight can help you respond positively to long term and emerging issues in the banking sector.
-
Capital markets
2020 is a demanding year for capital markets. Working with you, we're architecting the future of the sector.
-
Insurance
Our experienced expert team brings you technical expertise and insight to guide you through insurance sector challenges.
-
Investment management
Embracing innovation and shaping business models for long-term success.
-
Pensions
Pension provision is an essential issue for employers, and the role of the trustee is becoming increasingly challenging.
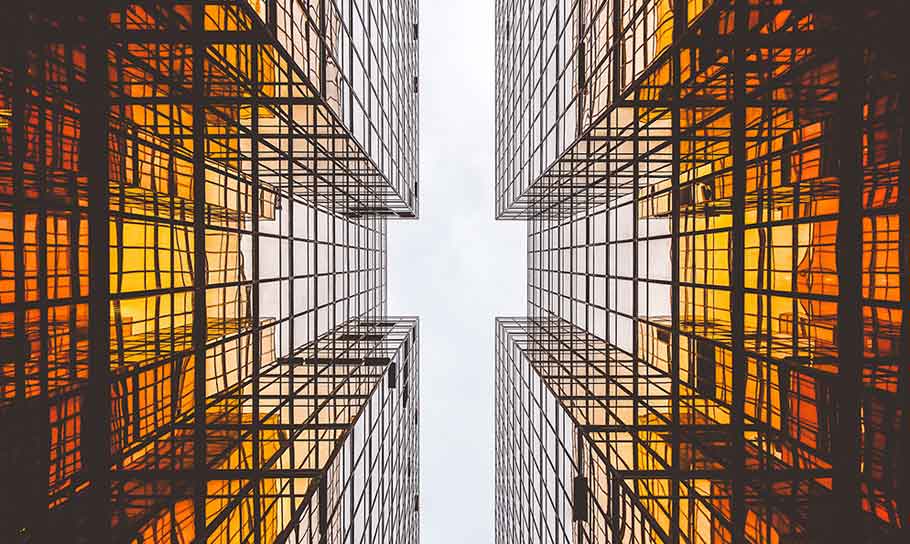
-
Central and devolved government
Helping central and devolved governments deliver change to improve our communities and grow our economies.
-
Infrastructure and transport
Delivering a successful transport or infrastructure project will require you to balance an often complex set of strategic issues.
-
Local government
Helping local government leverage technical and strategic expertise deliver their agendas and improve public services.
-
Regeneration development and housing
We provide commercial and strategic advice to assist your decision making in pursuing your objectives.
-
Health and social care
Sharing insight and knowledge to deliver transformation and improvement to health and social care services.
-
Charities
Supporting you to achieve positive change in the UK charity sector.
-
Education and skills
The education sector has rarely faced more risk or more opportunity to transform. You need to plan for the future.
-
Social housing
We are committed to helping change social housing for the better, and can help you make the most of every opportunity.
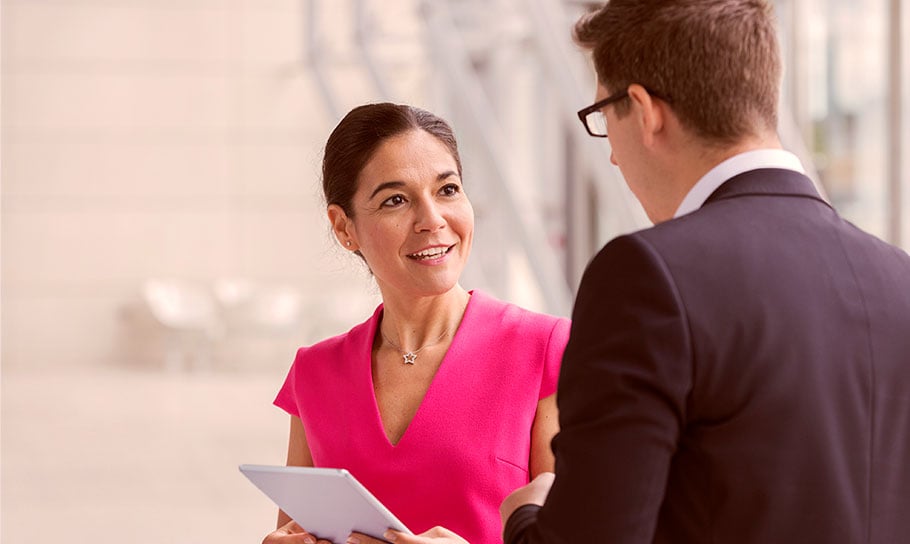
-
Technology
We work with dynamic technology companies of all sizes to help them succeed and grow internationally.
-
Telecommunications
Take all opportunities to realise your goals in telecommunications: from business refresh to international expansion.
-
Media
Media companies must stay agile to thrive in today’s highly competitive market – we’re here to support your ambitions.
In March 2023 it published a white paper setting its proposals on how it should regulate the use of artificial intelligence (AI) to ensure the UK can “create the right environment to harness the benefits of AI and remain at the forefront of technological developments … [while ensuring] the risks posed by AI can be addressed.” In March there were also high-profile public requests for developments of powerful AI solutions – such as ChatGPT and Bard - to be paused for at least six months to ensure that the impact of AI can be carefully “planned for and managed”.
Against this backdrop, Audit Committees are looking to their internal audit and technology risk functions to provide assurance that the risks associated with AI solutions are being appropriately managed.
What is AI and how are businesses using it?
Over the past 15-20 years we’ve seen a number of technological advancements impacting how organisations operate, disrupting the marketplace and creating new opportunities. AI is poised to be more disruptive than anything before it and many organisations are exploring how they can use AI to gain a competitive advantage and streamline their business processes.
Recently Google and OpenAI (heavily backed by Microsoft) launched Bard and ChatGPT, AI-powered chatbots that simulate human conversations and are capable of answering questions on almost any subject using natural language processing and machine learning. They can provide answers to fact-based questions (eg, who is the King of Norway?) through to more complex process-driven questions (eg, what are the steps I need to go through to plan and run a conference for 2000 people) and to creative questions (eg, tell me a short story about a pony).
John McCarthy, regarded by many as the ‘father of artificial intelligence’ stated that AI is simply “the science of making intelligent machines.” Today AI is a broad term that refers to everything from robotic process automation (RPA) to augmented intelligence solutions and cognitive decision making performed by software robots (bots). Many organisations are investing in AI research and technologies – such as neural networks, big data processing, data mining, robotics, machine learning, image recognition – to assist them in analysing data or making complex decisions in a manner that is faster and more accurate than humans. This can increase efficiencies and eliminate the need for more menial human tasks.
Examples of where businesses are deploying AI include:
- Automotive manufacturers developing self-driving vehicles
- Web chat bots as a first point of contact for their customers
- Using AI tools to filter CVs of potential candidates before being offered an interview
- Hotels using AI to make real time pricing updates to their rooms and prove ‘in-person’ customer services
- Logistics companies using AI tools to plan, and update, optimal route deliveries in real time
- Pharmaceutical organisations using AI to research drugs and diagnose illnesses in a fraction of the time it normally takes
- Retail stores using AI to automate elements of their supply chain and core financial processes
The challenges and risks associated with AI
Although AI provides an array of opportunities for businesses, there are a number of challenges that arise when implementing AI solutions. Audit Committees are increasingly looking to internal audit and technology risk functions to provide assurance that the risks associated with such strategies and solutions are being appropriately managed.
Key risk areas to consider include:
Strategy
With all the excitement around AI, organisations risk implementing AI solutions without a formal strategy and a valid business case, or don’t serve the organisation’s business objectives, ultimately wasting their resources.
Speed
Many organisations are rushing to adopt AI without first assessing the risks involved or controls required and currently there are very few frameworks or AI-specific regulations in place.
Knowledge
AI and its associated technologies are still relatively new and skills to design, implement, and manage AI solutions are scarce. Furthermore, employees will need to be trained to use the new AI solutions or redeployed into other areas of the business where their roles are made redundant by an AI solution.
Data
Fundamentally, AI systems rely on data. If the quality of the data is poor, or stored in separate and isolated systems, organisations may struggle to implement solutions to extract the value from their investment into AI.
Complexity
AI systems are inherently complex, relying on layers of code with multiple inputs and (at their purest) can learn and adapt without human intervention. If AI developments and solutions aren’t properly managed and monitored, they may make inaccurate (or even biased) decisions.
Governance
A lack of adequate governance arrangements over the development and deployment of AI may result in key business processes being undermined, breaches of company policy or delegated authorities, and unintended biases.
Ethics
There still remain a number of ethical concerns about using AI to make business decisions. Despite the shift in the public’s attitude towards using AI, some would still feel uncomfortable about being subject to this themselves. Individuals’ rights under regulation, such as GDPR, also need to be taken into account before a business adopts AI.
Looking forward: the growth of AI and challenge for internal audit and technology risk functions
The use of AI is expected to grow significantly over the coming years, with the global AI market size estimated to grow USD 1, 591 billion by 2030 (from USD 120 billion in 2022) (Precedence research). Research by IBM in 2022 indicated that 35% of companies were already using AI in their business, with an additional 42% stating they were exploring how to use AI, with adoption rates expected to only increase over the coming years.
Against this backdrop, audit committees are starting to charge their internal audit and technology risk teams to provide assurance over the risks associated with their organisation’s use of AI; the design, performance, monitoring and governance of AI based processes; and the programmes to implement AI solutions.
Assurance functions need to prepare themselves for how and when their business will deploy, or are already deploying, AI solutions. This will involve gaining an understanding of how AI operates and upskilling themselves so that they can provide advice and assurance to the business on the challenges and risks that AI brings. Given how quickly AI is evolving it will be critical that they can keep pace with developments in AI and continue to provide meaningful assurance.
This is posing a real challenge for internal audit and technology risk functions as many aren’t well equipped to provide the assurance that their audit committees and board are looking for. This is even more critical now, given that a number of organisations are only just starting to embark on their AI journey.
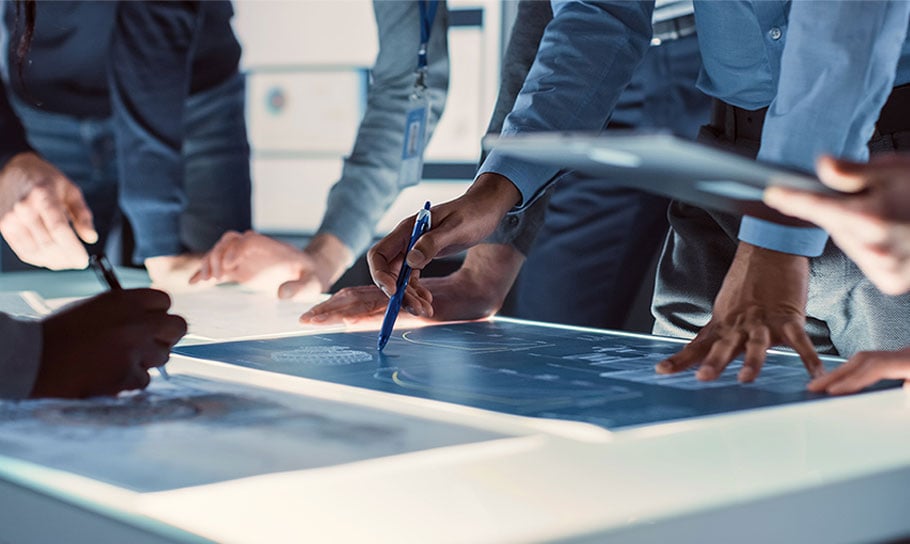
An approach to auditing AI
Internal audit and technology risk teams need to adopt an approach to auditing AI that provides assurance that the risks associated with this are being managed while not hindering their business’s ability to realise the opportunities that AI presents. Areas that internal audit teams may wish to consider are:
Strategy and governance
It’s key that an organisation’s use of AI has a valid business case and strategy, and supports the wider business’ corporate objectives strategy and corporate objectives, and the programmes to roll out of AI are appropriately governed. It’s equally important that organisations’ boards and/or senior management teams implement appropriate structures, processes and procedures to direct, manage and monitor the use of AI across the organisation. As part of this, adequate communication plans should be in place to ensure stakeholders are made aware of how the organisation intends to use AI and plans should be in place to help re-train employees whose roles will be disrupted (or replaced) by AI solutions. Arrangements should also be in place to respond to feedback, questions and complaints from customers regarding how their data is subject to AI processing.
AI technology control environment
All AI solutions and the data they rely upon reside in infrastructure – whether that’s hosted internally or externally in the cloud. This infrastructure needs to be properly managed, maintained and secured. In addition, the AI solutions themselves need to be appropriately administered so that unauthorised individuals don’t obtain access to them. The teams that support the AI infrastructure and solutions also need to be provided training to fill any AI skills gaps. In addition, any third parties involved in hosting or supporting AI infrastructure or solutions need to be proactively managed.
Data governance and quality
All AI is underpinned by data. If the quality of this data is poor, the data isn’t sufficiently maintained, or disparate systems holding data aren’t well integrated, this could lead to difficulties in implementing an AI solution or invalid decisions being made based on the AI output. To enable the implementation of an AI solution, most organisations will need to transform their data environment. Such ‘big data’ strategies will need to be carefully planned, aligned to the business objectives and monitored to ensure that they deliver the intended value.
AI model development, monitoring and maintenance
The development of AI solutions must be formally managed and controlled, even in Agile environments, to ensure they deliver the intended results and meet the required quality levels. Process mapping exercises should be performed, formally reviewed and approved as part of these processes, to ensure the models are truly aligned to business processes and support company policies. Where possible, pilot studies should be performed to assess the impacts of AI before a full rollout. Exception-handling routines should be established to manage situations when an AI model’s activities fall outside of the expected operating parameters In addition, where possible, natural language processing should be used to make the solution appear human to the user. Access to source code and development arrangements should be carefully administered to help prevent inappropriate changes from being made.
Ethics and human bias
AI developers should pay careful attention to this when developing and testing solutions (including the data and models underpinning these) to ensure they’re free from human bias. The ethics of using AI to help make decisions should be considered before adopting a solution, and all AI decisions should be fully transparent and auditable. Furthermore, all those who are subject to AI processes should be made aware of this in accordance with the GDPR, and their data shouldn’t be processed, by AI or otherwise, for any other reasons than for the purpose it was originally obtained for.
The use of AI by businesses is only expected to increase over the coming years If internal audit functions don’t start upskilling themselves and acting now, their organisation may not be adequately prepared to manage the risks, and realise the opportunities, associated with AI. Alternatively they may find their organisations are some way down their AI journey before they realise that they have set off in the wrong direction without laying the foundations in place.
To further discuss the risks associated with AI and how your internal audit and second line technology risks teams can provide assurance over these, get in touch with James Durrant.
Get the latest insights, events and guidance, straight to your inbox.