-
Governance advisory
We guide boards and management teams in frameworks, team processes and leadership dynamics to deliver sustainable value.
-
Financial services advisory
Get market-driven expertise to achieve your goals in banking, insurance, capital markets, and investment management.
-
Business risk services
Our market-driven expertise helps firms keep growing and manage risk in an evolving regulatory landscape.
-
Risk
Meet risks with confidence and transform your business – we support you to manage risk and deliver on your goals.
-
Economic consulting
Bespoke guidance grounded in complex economic theory and practical sector insight to help you make the right decisions.
-
Government and public sector
Experience and expertise in delivering quality public sector advisory and audits.
-
Business consulting
Partnering with you to deliver sustainable business change that helps you realise your ambitions.
-
Transaction advisory services
Whether buying or selling, we help you get the deal done with our comprehensive range of transaction advisory services.
-
Financial accounting advisory services (FAAS)
Our FAAS team can support your finance function with the flexible resource they need to get results.
-
Corporate finance advisory
Building a business is never easy. We help you maximise the value of your business and find the right option.
-
Valuations
Help to understand or support the valuation of a business or asset.
-
Insolvency and global asset recovery
We provide asset tracing and seamless cross-border global recovery for clients.
-
Forensic and investigation services
Market-driven expertise in investigations, dispute resolution and digital forensics.
-
Restructuring
Our restructuring team help lenders, investors and management navigate contingency plans, restructuring and insolvency.
-
Transformation consulting
Is business transformation a priority for your organisation? Our expert insight and guidance can help you achieve it.
-
Pensions assurance
A tailored service that responds to evolving risks and regulations.
-
Accounting services
Optimise your growth with expert accounting services. Contact us today.
-
Royalty and intellectual property (IP) audits
Enhance IP asset protection with our royalty and IP audit services. Expertise in licensing, revenue detection, and compliance improvements.
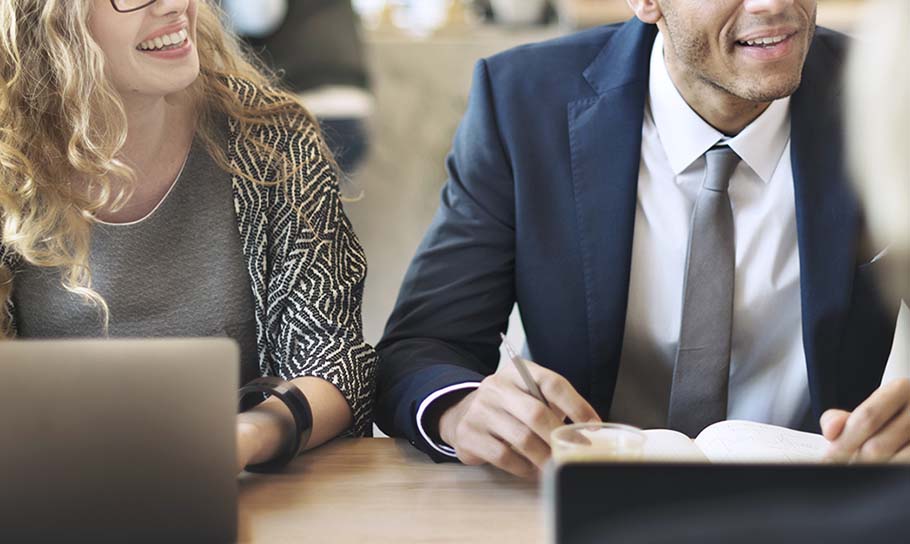
-
Business consulting
Partnering with you to deliver sustainable business change that helps you realise your ambitions.
-
Corporate Simplification
Release value, reduce compliance complexity, and improve tax efficiency by streamlining your group structure.
-
Economic consulting
Bespoke guidance grounded in complex economic theory and practical sector insight to help you make the right decisions.
-
Financial accounting advisory services (FAAS)
Our FAAS team can support your finance function with the flexible resource they need to get results.
-
Governance advisory
We guide boards and management teams in frameworks, team processes and leadership dynamics to deliver sustainable value.
-
International
Unlock global opportunities with our local expertise and worldwide reach.
-
People advisory
Driving business performance through people strategy and culture.
-
Strategy Group
Successful business strategy is rooted in a clear understanding of the market, customer segmentation and how purchase decisions vary.
-
Respond: Data breach, incident response and computer forensics
Are you prepared for a cyber failure? We can help you avoid data breaches and offer support if the worst happens.
-
Comply: Cyber security regulation and compliance
Cyber security regulation and compliance is constantly evolving. Our team can support you through the digital landscape.
-
Protect: Cyber security strategy, testing and risk assessment
Cyber security threats are constantly evolving. We’ll work with you to develop and test robust people, process and technology defences to protect your data and information assets.
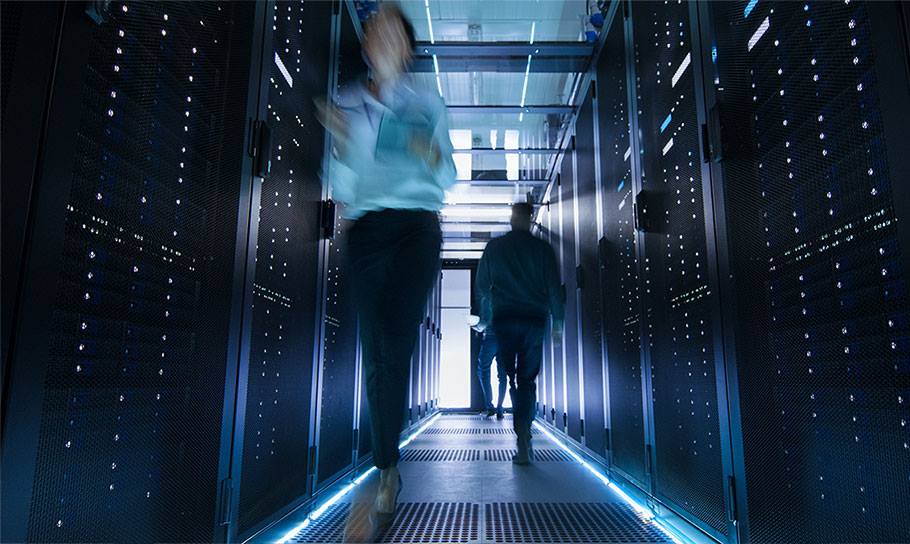
-
Corporate finance advisory
Building a business is never easy. We help you maximise the value of your business and find the right option.
-
Debt advisory
Working with borrowers and private equity financial sponsors on raising and refinancing debt. We can help you find the right lender and type of debt products.
-
Financial accounting advisory services (FAAS)
Our FAAS team can support your finance function with the flexible resource they need to get results.
-
Financial modelling services
Financial modelling that helps you wrestle with your most pressing business decisions.
-
Operational deal services
Enabling transaction goals through due diligence, integration, separation, and other complex change.
-
Our credentials
Search our transactions to see our experience in your sector and explore the deals advisory services we've delivered.
-
Transaction advisory services
Whether buying or selling, we help you get the deal done with our comprehensive range of transaction advisory services.
-
Valuations
Help to understand or support the valuation of a business or asset.
-
The ESG agenda
Shape your ESG agenda by identifying the right metrics, sustainable development and potential business value impact.
-
ESG driven business transition
Whatever your ESG strategy, we can support your organisation as it evolves while maximising efficiency and profitability.
-
ESG programme and change management
Do you have the right capabilities to drive the delivery of your ESG strategy to realise your targets?
-
ESG risk management
You must protect, comply, understand and influence to successfully manage the risk involved with ESG issues. We can help.
-
ESG strategy, risk and opportunity identification
We can help you clearly define your ESG Strategy, with the risks and opportunities identified and managed.
-
Create value through effective ESG communication
Building trust and engagement with your stakeholders on your ESG strategy.
-
ESG metrics, targets and disclosures
The pressure to report your ESG progress is growing. Do your targets measure up?
-
ESG governance, leadership and culture framework
Make the most of ESG opportunities by effectively embedding your strategy across your organisation.
-
ESG and non-financial assurance
Support your board to be confident in supplying robust information that withstands scrutiny.
-
Transition planning to net zero
Supporting your organisation in the transition to net zero.
-
Actuarial and insurance consulting
We consult extensively to the life insurance, general insurance, health insurance and pensions sectors.
-
Business risk services
Our market-driven expertise helps firms keep growing and manage risk in an evolving regulatory landscape.
-
Financial crime
Helping you fight financial crime in a constantly changing environment
-
Financial services business consulting
Leverage our diverse capabilities to manage challenges and take opportunities: from assurance to transformation
-
Financial services tax
Helping financial services firms navigate the global financial services and funds tax landscape.
-
Regulatory and compliance
Providing an exceptional level of regulatory and compliance to firms across the financial services industry.
-
Corporate intelligence
Corporate intelligence often involves cross-border complexities. Our experienced team can offer support.
-
Litigation support
Industry-wide litigation support and investigation services for lawyers and law firms.
-
Disputes advisory
Advising on quantum, accounting and financial issues in commercial disputes.
-
Forensic investigations and special situations
Do you need clarity in an uncertain situation? If you're accused of wrongdoing we can help you get the facts right.
-
Forensic data analytics
Our forensic data analytics team are helping businesses sift the truth from their data. See how we can help your firm.
-
Monitoring trustee and competition services
Monitoring trustee services to competition, financial and regulatory bodies.
-
Financial crime
Supporting your fight against financial crime in an ever-changing environment
-
Public sector advisory
To deliver excellent public services, local and central government need specialist support.
-
Public sector consulting
Helping public sector organisations maintain oversight of services and understand what's happening on the ground.
-
Public sector audit and assurance
As a leading UK auditor, we have unparalleled insights into the risks, challenges and opportunities that you face.
-
Contentious estates and family disputes
We manage complex and sensitive disputes through to resolution.
-
Digital Asset Recovery
Get guidance and technical expertise on digital finance and cryptoasset recovery from our dedicated crypto hub.
-
Grant Thornton Offshore
Grant Thornton Offshore is our one-stop global solution for insolvency, asset recovery, restructuring and forensics services.
-
Insolvency Act Portal
Case information and published reports on insolvency cases handled by Grant Thornton UK LLP.
-
Litigation support
Industry-wide litigation support and investigation services for lawyers and law firms.
-
Personal insolvency
We can support you to maximise personal insolvency recovery and seek appropriate debt relief.
-
South Asia business group
Supporting your growth in the UK-India economic corridor and beyond.
-
US business group
Optimise your trans-Atlantic operations with local knowledge and global reach.
-
Japan business group
Bridging the commercial and cultural divide and supporting your ambitions across Japan and the UK.
-
Africa business group
Connecting you to the right local teams in the UK, Africa, and the relevant offshore centres.
-
China-Britain business group
Supporting your operations across the China – UK economic corridor.
-
Asset based lending advisory
Helping lenders, their clients and other stakeholders navigate the complexities of ABL.
-
Contingency planning and administrations
In times of financial difficulty, it is vital that directors explore all the options that are available to them, including having a robust ‘Plan B’.
-
Corporate restructuring
Corporate restructuring can be a difficult time. Let our team make the process simple and as stress-free as possible.
-
Creditor and lender advisory
Whether you're a creditor or lender, complex restructurings depend on pragmatic commercial advice
-
Debt advisory
Our debt advisory team can find the right lender to help you in restructuring. Find out how our experts can support you.
-
Financial services restructuring and insolvency
Financial services restructuring and insolvency is a competitive marketplace. Our team can help you navigate this space.
-
Pensions advisory services
DB pension-schemes need a balanced approach that manages risk for trustees and sponsors in an uncertain economy.
-
Restructuring and insolvency tax
Tax will often be crucial in a plan to restructure a distressed business. Our team can guide you through the process.
-
Restructuring Plans
Market leading experience in advising companies and creditors in Restructuring Plan processes.
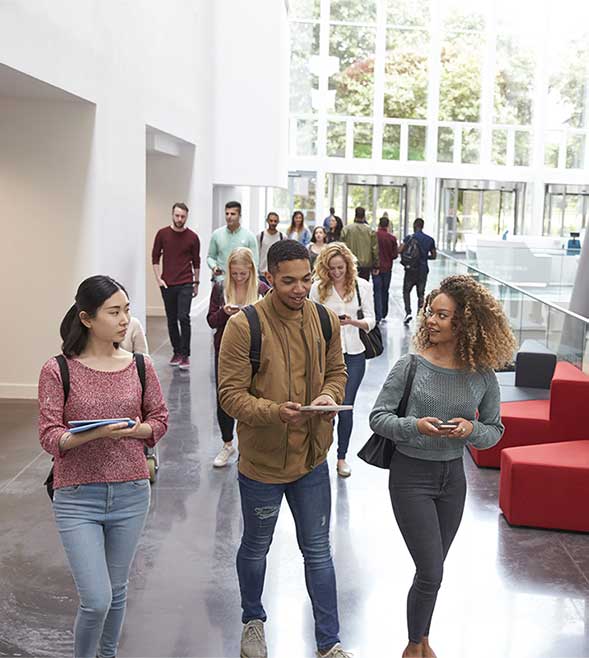
-
Controls advisory
Build a robust internal control environment in a changing world.
-
Data assurance and analytics
Enhancing your data processes, tools and internal capabilities to help you make decisions on managing risk and controls.
-
Enterprise risk management
Understand and embrace enterprise risk management – we help you develop and connect risk thinking to your objectives.
-
Internal audit services
Internal audit services that deliver the value and impact they should.
-
Managing risk and realising ESG opportunities
Assess and assure risk and opportunities across ESG with an expert, commercial and pragmatic approach.
-
Project, programme, and portfolio assurance
Successfully delivering projects and programmes include preparing for the wider impact on your business.
-
Service organisation controls report
Independent assurance provides confidence to your customers in relation to your services and control environment.
-
Supplier and contract assurance
Clarity around key supplier relationships: focusing on risk, cost, and operational performance.
-
Technology risk services
IT internal audits and technology risk assurance projects that help you manage your technology risks effectively.
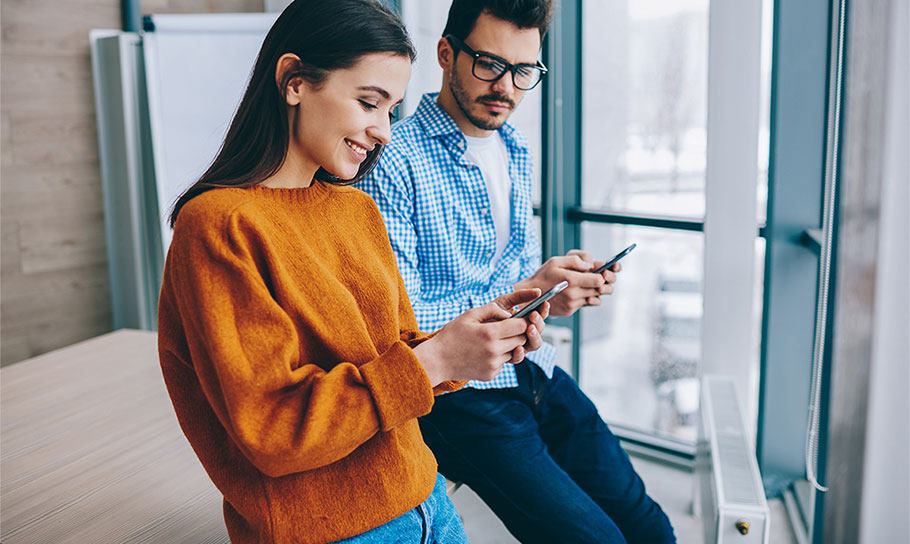
-
Capital allowances (tax depreciation)
Advisory and tools to help you realise opportunities in capital allowances.
-
Corporate tax
Helping companies manage corporate tax affairs: delivering actionable guidance to take opportunities and mitigate risk.
-
Employer solutions
We will help you deliver value through your employees, offering pragmatic employer solutions to increasing costs.
-
Indirect tax
Businesses face complex ever changing VAT regimes, guidance and legislation. We can help you navigate these challenges.
-
International tax
Real-world international tax advice to help you navigate a changing global tax landscape.
-
Our approach to tax
We advise clients on tax law in the UK and, where relevant, other jurisdictions.
-
Private tax
Tax experts for entrepreneurs, families and private business. For now and the long term.
-
Real estate tax
Stay ahead of real estate tax changes with holistic, tax-efficient solutions.
-
Research and development tax incentives
We can help you prepare optimised and robust research and development tax claims.
-
Tax dispute resolution
We make it simple to stay compliant and avoid HMRC tax disputes
-
Tax risk management
We work with you to develop effective tax risk management strategies.
-
Skills and training
Get the right support to deliver corporate and vocational training that leads the way in an expanding market.
-
Private education
Insight and guidance for all businesses in the private education sector: from early years to higher education and edtech.
-
Facilities management and property services
Get insight and strategic support to take opportunities that protect resilience and drive UK and international growth.
-
Recruitment
Helping recruitment companies take opportunities to achieve their goals in a market where talent and skills are key.
-
Food and beverage (F&B)
We can help you find the right ingredients for growth in your food and beverage business.
-
Travel, tourism and leisure
Tap into our range of support for travel, tourism and leisure businesses in this period of challenge and change.
-
Retail, e-commerce and consumer products
With multiple challenges and opportunities in the fast-evolving retail sector, make sure you are ready for them.
-
Banking
Our expertise and insight can help you respond positively to long term and emerging issues in the banking sector.
-
Capital markets
2020 is a demanding year for capital markets. Working with you, we're architecting the future of the sector.
-
Insurance
Our experienced expert team brings you technical expertise and insight to guide you through insurance sector challenges.
-
Investment management
Embracing innovation and shaping business models for long-term success.
-
Pensions
Pension provision is an essential issue for employers, and the role of the trustee is becoming increasingly challenging.
-
Payments advisory and assurance
Payment service providers need to respond to rapidly evolving technical innovations and increased regulatory scrutiny.
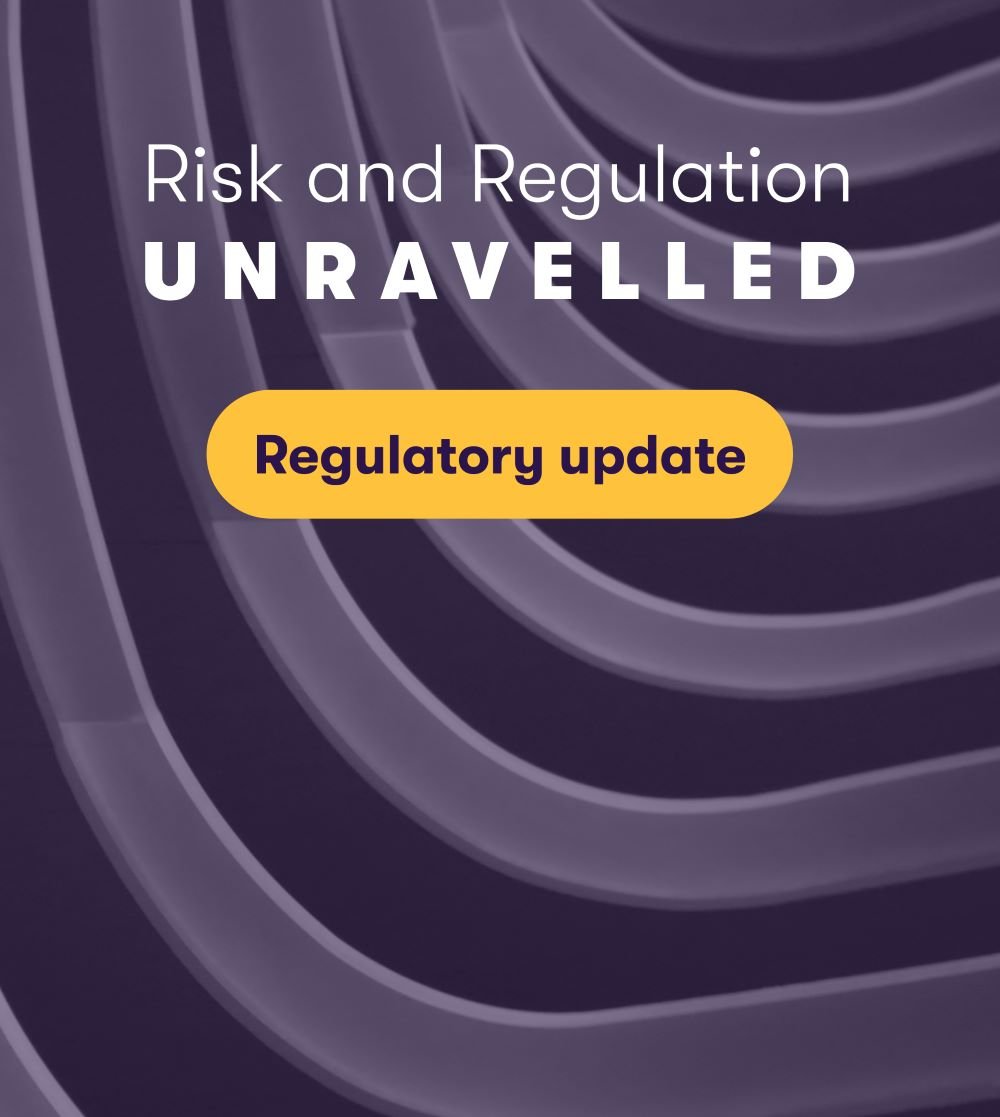
-
Central and devolved government
Helping central and devolved governments deliver change to improve our communities and grow our economies.
-
Infrastructure and transport
Delivering a successful transport or infrastructure project will require you to balance an often complex set of strategic issues.
-
Local government
Helping local government leverage technical and strategic expertise deliver their agendas and improve public services.
-
Regeneration development and housing
We provide commercial and strategic advice to assist your decision making in pursuing your objectives.
-
Health and social care
Sharing insight and knowledge to deliver transformation and improvement to health and social care services.
-
Charities
Supporting you to achieve positive change in the UK charity sector.
-
Education and skills
The education sector has rarely faced more risk or more opportunity to transform. You need to plan for the future.
-
Social housing
We are committed to helping change social housing for the better, and can help you make the most of every opportunity.
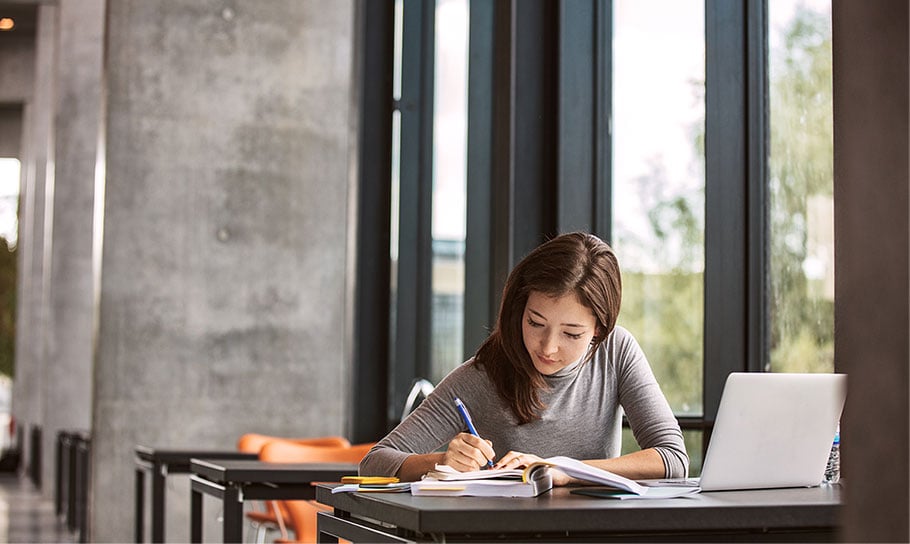
-
Technology
We work with dynamic technology companies of all sizes to help them succeed and grow internationally.
-
Telecommunications
Take all opportunities to realise your goals in telecommunications: from business refresh to international expansion.
-
Media
Media companies must stay agile to thrive in today’s highly competitive market – we’re here to support your ambitions.
Artificial intelligence (AI) can be a huge asset in risk and assurance: increasing accuracy and reliability in generating insights, streamlining processes to increase productivity, and enhancing quality. It can add value to your organisation by creating new ways of working and opening growth opportunities.
The potential applications for using AI in your own operations depend on your specific needs and business context. The key concern, however, will always be security of the information used by AI tools and we have noted our precautions below. As the full risks of AI are still being understood, it's up to every organisation to assess their own possible exposures.
1 Natural language processing in data analysis
Data can have various types and formats, such as written language in documents, tables, spreadsheets, or spoken language, recordings or transcripts. It can be hard to gather different kinds of data and examine them effectively every day for many people. By using powerful technologies, natural language processing (NLP) can process diverse and complex data formats and produce useful inputs for AI tools to use. NLP can help increase business value by enhancing the quality of reviews and audits, by organising, summarising, and analysing data.
There are several ways to apply intelligent document analysis techniques using NLP, including:
With optical character recognition (OCR), large amounts of documents such as contracts, invoices, and reports can be transformed from scanned images to text and sorted by NLP based on specified topics and criteria. Unstructured data like emails, images, and texts can be easily handled without manually reviewing the documents. The information extracted is then used to verify the correctness and frequency of transactions under review without spending a lot of time on document examination.
NLP can also help to find similarities in documents. It can mark similarities and possible duplicates based on the key features it identifies. This makes it easier to review documents and select corroborating files for audit evidence.
Large language models (LLMs) are a form of AI that can imitate human intelligence, can handle large amounts of data such as transaction history, recorded meetings or inventory levels. They are useful when data is unstructured i.e. plain text or media posts. AI tools can then let you query your data and give an instant answer. The incorporation of these kinds of insights from NLP analysis into risk management processes, with human supervision, can enable prompt actions and improve your assurance capability.
2 Predictive analytics
Predictive analytics uses machine learning to extract useful trends, patterns, and behaviour from historical datasets. It can provide deep insights into key risk indicators. With data mining and statistics, predictive analytics can help with risk assessment and testing of controls. It can also reveal current and future risks, and help prevent major problems before they happen.
Risk and assurance teams can leverage this capability to fully optimise the use of data by incorporating predictive analytics in the assessment of fraud. Combining the function to process large volumes of unstructured data with machine learning can detect unusual patterns and behaviours to identify fraud. This can help determine unusual behaviours and suspicious risk profiles from extensive data sets to trigger investigation.
We've developed a flexible solution embedded with predictive analytics for critical business processes.
3 Risk intelligence
AI can improve the quality and precision of vital risk information by analysing data automatically and extensively. AI can assist risk and assurance teams by collecting and integrating data from internal and external sources, providing a wider perspective of the whole enterprise.
These are some of the best ways that AI can be used to develop risk intelligence.
In addition to using machine learning for adverse event prediction as mentioned above, large amounts of data from different sources can be used to describe critical adverse situations that can harm the organisation. These sources could include social media, news articles and cyber alerts. Companies can use AI to rapidly detect possible threats and examine patterns and trends in the data.
Contextual data can help with comparing risks and ranking them by importance. Companies can use AI to give scores to the possible threats that they identify, and use these scores to decide which risks need more attention and resources.
4 Researching content for audit programmes
Developing an audit programme is a crucial part of any audit engagement. By using Large Language Models (LLMs), such as ChatGPT or Microsoft Co-Pilot, the process can be more efficient - reducing time and resources by automating tasks. Auditors can use it for research and to enhance their audit plans to suit their particular scope and objectives. However, this is only a preliminary step, co-source expertise and SME knowledge is needed for customisation and best practice.
ChatGPT can produce a simple P2P control audit plan that covers P2P control audit goals, range, risk analysis, control review, testing methods, results, suggestions, reporting, and follow-up actions. The format ensures a comprehensive control assessment but will need adjustment for different organisations.
Benefits of using LLMs to assist with audit programme development
- Provide valuable learning opportunities for less experienced auditors – junior auditors can leverage AI tools to save time and expedite knowledge acquisition, enhancing their skill sets
- Access to information, guidance, and support auditors’ professional development and expertise in the auditing field
Precautions: auditors need to be mindful of a few cautionary points while using AI solutions
- Public AI uses the data provided by users for further learnings – so, auditors should be cautious not to provide sensitive corporate or client information
- If AI solutions are implemented in-house, auditing functions need to ensure that the systems are trained well using adequate datasets and that the training is continuous
- Review and adjust AI-generated audit programmes to align with the specific goals and scope of the audit
- Exercise due diligence in using LLM tools for internal audit, avoiding negligence in favour of convenience
5 Reviewing code
By using AI, auditors can examine computer code faster and more reliably than by checking it manually. This is useful for internal audits of important processes or automated controls that involve large or complicated code repositories. AI can support in more ways, such as:
AI tools can automatically scan and analyse code to find security issues, mistakes, or detect patterns that indicate potential issues. This helps auditors to efficiently address weaknesses, making their work more productive, allowing them to focus on more important tasks.
AI can verify code against coding rules, laws, or internal policies to uphold compliance. By doing this regularly, AI enhances the precision of detecting coding defects, risks, and compliance matters with the code. This is beneficial for complex or challenging codes, as it helps auditors efficiently spot potential security problems, coding errors, or deviations from compliance requirements.
AI-enabled applications can constantly scan code repositories (systems that store and control computer code), notifying auditors of any alterations, security risks, or policy breaches as they happen.
Watch the video to see how we can help
For more insight and guidance, get in touch with Alex Hunt and Nikhil Asthana.
Get the latest insights, events and guidance, straight to your inbox.